The architecture of BERT
In Chapter 2, Getting Started with the Architecture of the Transformer Model, we defined the building blocks of the architecture of the Original Transformer. Think of the Original Transformer as a model built with LEGO® bricks. The construction set contains bricks such as encoders, decoders, embedding layers, positional encoding methods, multi-head attention layers, masked multi-head attention layers, post-layer normalization, feed-forward sub-layers, and linear output layers.
The bricks come in various sizes and forms. As a result, you can spend hours building all sorts of models using the same building kit! Some constructions will only require some of the bricks. Other structures will add a new piece, like when we obtain additional bricks for a model built using LEGO® components.
BERT introduces bidirectional attention to transformer models. Bidirectional attention requires many other changes to the Original Transformer model.
We will not go through the building blocks of transformers described in Chapter 2, Getting Started with the Architecture of the Transformer Model. You can consult Chapter 2 at any time to review an aspect of the building blocks of transformers. Instead, this section will focus on the specific aspects of BERT models.
We will focus on the evolutions designed by Devlin et al. (2018), which describe the encoder stack. We will first go through the encoder stack and then the preparation of the pretraining input environment. Then, we will explain the two-step framework of BERT: pretraining and fine-tuning.
Let’s first explore the encoder stack.
The encoder stack
The first building block we will take from the Original Transformer model is an encoder layer. The encoder layer, as described in Chapter 2, Getting Started with the Architecture of the Transformer Model, is shown in Figure 5.1:
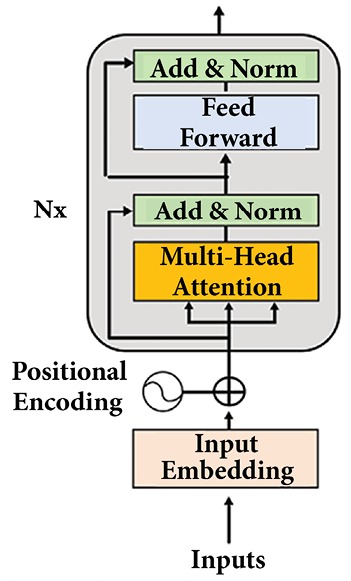
Figure 5.1: The encoder layer
The BERT model does not use decoder layers. A BERT model has an encoder stack but no decoder stacks. The BERT model uses Masked Language Modeling (MLM) in which some input tokens are hidden (“masked”), and the attention layers must learn to understand the context. The model will predict the hidden tokens, as we will see when we zoom into a BERT encoder layer in the following sections.
The Original Transformer contains a stack of N = 6 layers. The number of dimensions of the Original Transformer is dmodel = 512. The number of attention heads of the Original Transformer is A = 8. The dimensions of the head of the Original Transformer are:
BERT encoder layers are larger than the Original Transformer model.
Different sizes of BERT models can be built with the encoder layers:
- BERTBASE, which contains a stack of N = 12 encoder layers. dmodel = 768, which can also be expressed as H = 768, as in the BERT paper. A multi-head attention sub-layer contains A = 12 heads. The dimension of each head zA remains 64 as in the Original Transformer model:
The output of each multi-head attention sub-layer before concatenation will be the output of the 12 heads:
output_multi-head_attention={z0, z1, z2,…, z11}
- BERTLARGE, which contains a stack of N = 24 encoder layers. dmodel = 1024. A multi-head attention sub-layer contains A = 16 heads. The dimension of each head zA also remains 64 as in the Original Transformer model:
The output of each multi-head attention sub-layer before concatenation will be the output of the 16 heads:
output_multi-head_attention={z0, z1, z2,…, z15}
The sizes of the models can be summed up as follows:
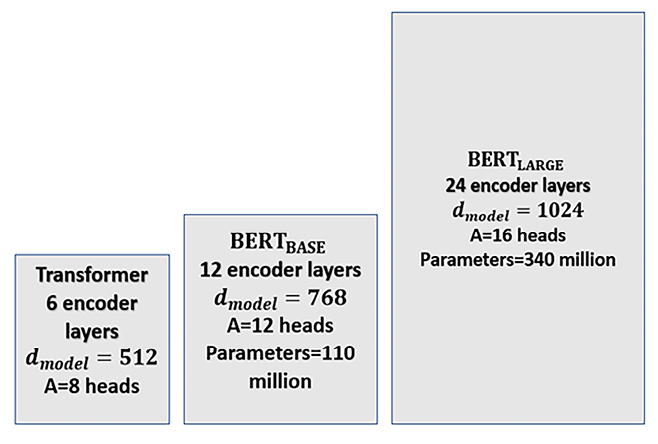
Figure 5.2: Transformer models
BERT models are not limited to these configurations, which illustrate the main aspects of BERT models. Numerous variations are possible.
Size and dimensions play an essential role in BERT-style pretraining. BERT models are like humans; they produce better results with more working memory (dimensions) and knowledge (data). Large transformer models that learn large amounts of data will pretrain better for downstream NLP tasks.
Let’s go to the first sub-layer and see the fundamental aspects of input embedding and positional encoding in a BERT model.
Preparing the pretraining input environment
The BERT model only has encoder layers and no decoder stack. Its architecture features a multi-head self-attention mechanism that allows each token to learn to understand all the surrounding tokens (masked or not). This bidirectional method enables BERT to understand the context on both sides of each token.
A masked multi-head attention layer masks some tokens randomly to force this system to learn contexts. For example, take the following sentence:
The cat sat on it because it was a nice rug.
If we have just reached the word it
, the input of the encoder could be:
The cat sat on it<masked sequence>
The random mask can be anywhere in the sequence, not necessarily at the end. To know what it
refers to, we need to see the whole sentence to reach the word rug
and figure out that it
was the rug.
The authors enhanced their bidirectional attention model, letting an attention head attend to all the words from left to right and right to left. In other words, the self-attention mask of an encoder could do the job without being hindered by the masked multi-head attention sub-layer of the decoder.
The model was trained with two tasks. The first method is MLM. The second method is Next-Sentence Prediction (NSP).
Let’s start with MLM.
Masked language modeling
MLM does not require training a model with a sequence of visible words followed by a masked sequence to predict.
BERT introduces the bidirectional analysis of a sentence with a random mask on a word of the sentence.
It is important to note that BERT applies WordPiece
, a subword segmentation tokenization method, to the inputs. It also uses learned positional encoding, not the sine-cosine approach.
A potential input sequence could be:
The cat sat on it because it was a nice rug.
The decoder could potentially mask the attention sequence after the model reaches the word it
:
The cat sat on it <masked sequence>.
But the BERT encoder masks a random token to make a prediction, which makes it more powerful:
The cat sat on it [MASK] it was a nice rug.
The multi-attention sub-layer can now see the whole sequence, run the self-attention process, and predict the masked token.
The input tokens were masked in a tricky way to force the model to train longer but produce better results with three methods:
- Surprise the model by not masking a single token on 10% of the dataset; for example:
The cat sat on it [because] it was a nice rug.
- Surprise the model by replacing the token with a random token on 10% of the dataset; for example:
The cat sat on it [often] it was a nice rug.
- Replace a token with a
[MASK]
token on 80% of the dataset; for example:
The cat sat on it [MASK] it was a nice rug.
The authors’ bold approach avoids overfitting and forces the model to train efficiently.
BERT was also trained to perform next-sentence prediction.
Next-sentence prediction
The second method invented to train BERT is NSP. The input contains two sentences. In 50% of the cases, the second sentence was the actual second sentence of a document. In 50% of the cases, the second sentence was selected randomly and had no relation to the first one.
Two new tokens were added:
[CLS]
is a binary classification token added to the beginning of the first sequence to predict if the second sequence follows the first sequence. A positive sample is usually a pair of consecutive sentences taken from a dataset. A negative sample is created using sequences from different documents.
[SEP]
is a separation token that signals the end of a sequence, such as a sentence, sentence part, or question, depending on the task at hand.
For example, the input sentences taken out of a book could be:
The cat slept on the rug. It likes sleeping all day.
These two sentences will become one complete input sequence:
[CLS] the cat slept on the rug [SEP] it likes sleep ##ing all day[SEP]
This approach requires additional encoding information to distinguish sequence A from sequence B.
Note that the double hash (##) is because of the WordPiece tokenization used. The word “sleep” was tokenized separately from “ing.” This enables the tokenizer to work on a smaller dictionary of subwords, WordPiece, and then assemble them through the training process.
If we put the whole embedding process together, we obtain the following:
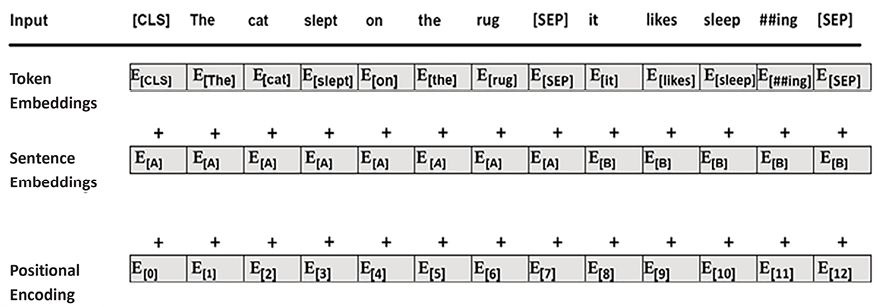
Figure 5.3: Input embeddings
The input embeddings are obtained by summing the token embeddings, the segment (sentence, phrase, word) embeddings, and the positional encoding embeddings.
The input embedding and positional encoding sub-layer of a BERT model can be summed up as follows:
- A sequence of words is broken down into
WordPiece
tokens. - A
[MASK]
token will randomly replace the initial word tokens for MLM training. - A
[CLS]
classification token is inserted at the beginning of a sequence for classification purposes. - A
[SEP]
token separates two sentences (segments, phrases) for NSP training:- Sentence embedding is added to token embedding, so that sentence A has a different sentence embedding value than sentence B.
- Positional encoding is learned. The sine-cosine positional encoding method of the Original Transformer is not applied.
Some additional key features of BERT are:
- It uses bidirectional attention in its multi-head attention sub-layers, opening vast horizons of learning and understanding relationships between tokens.
- It introduces scenarios of unsupervised embedding and pretraining models with unlabeled text. Unsupervised methods force the model to think harder during the multi-head attention learning process. This makes BERT learn how languages are built and apply this knowledge to downstream tasks without having to pretrain each time.
- It also uses supervised learning, covering all bases in the pretraining process.
BERT has improved the training environment of transformers. Let’s now see the motivation for pretraining and how it helps the fine-tuning process.
Pretraining and fine-tuning a BERT model
BERT is a two-step framework. The first step is pretraining, and the second is fine-tuning, as shown in Figure 5.4:
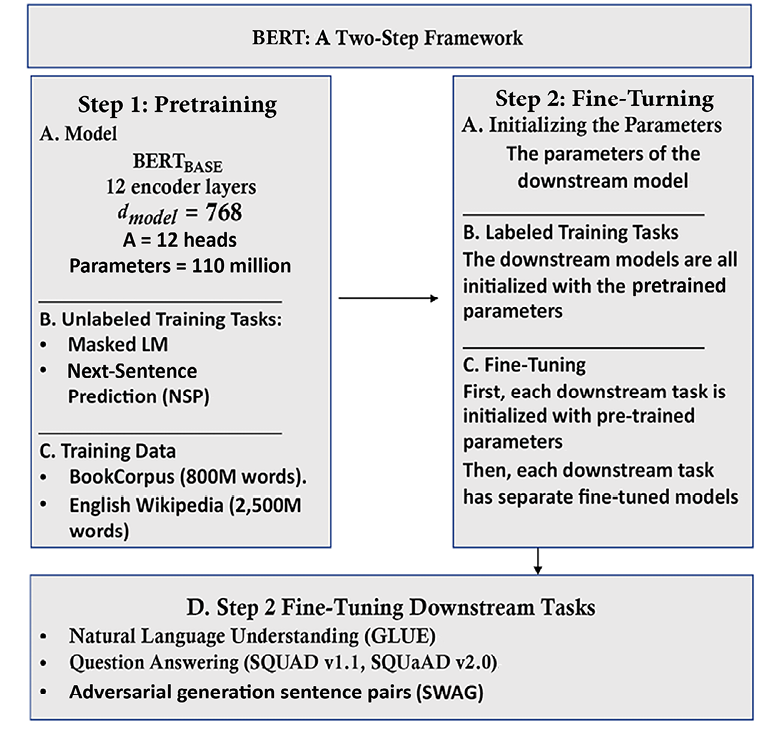
Figure 5.4: The BERT framework
Training a transformer model can take hours, if not days. Therefore, it takes quite some time to engineer the architecture and parameters and select the proper datasets to train a transformer model.
Pretraining is the first step of the BERT framework, which can be broken down into two sub-steps:
- Defining the model’s architecture: number of layers, number of heads, dimensions, and the other building blocks of the model.
- Training the model on MLM and NSP tasks.
The second step of the BERT framework is fine-tuning, which can also be broken down into two sub-steps:
- Initializing the downstream model chosen with the trained parameters of the pretrained BERT model
- Fine-tuning the parameters for specific downstream tasks such as Recognizing Textual Entailment (RTE), question answering (
SQuAD v1.1
,SQuAD v2.0
), and Situations With Adversarial Generations (SWAG).
In this chapter, the BERT model we will fine-tune will be trained on the Corpus of Linguistic Acceptability (CoLA). The downstream task is based on the Neural Network Acceptability Judgments paper by Alex Warstadt, Amanpreet Singh, and Samuel R. Bowman.
We will fine-tune a BERT model that will determine the grammatical acceptability of a sentence. The fine-tuned model will have acquired a certain level of linguistic competence.
We have gone through BERT’s architecture and its pretraining and fine-tuning framework. Let’s now fine-tune a BERT model.