Asynchronous n-step Q-learning
The architecture of asynchronous n-step Q-learning is, to an extent, similar to that of asynchronous one-step Q-learning. The difference is that the learning agent actions are selected using the exploration policy for up to
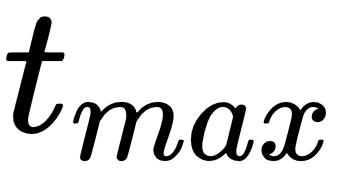
 steps or until a terminal state is reached, in order to compute a single update of policy network parameters. This process listsÂ
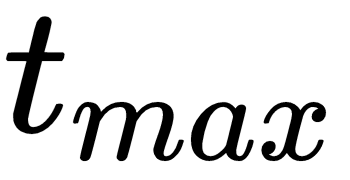
 rewards from the environment since its last update. Then, for each time step, the loss is calculated as the difference between the discounted future rewards at that time step and the estimated Q-value. The gradient of this loss with respect to thread-specific network parameters for each time step is calculated and accumulated. There are multiple such learning agents running and accumulating the gradients in parallel. These accumulated gradients are used to perform asynchronous updates of policy network parameters.
The pseudo-code for asynchronous n-step Q-learning is shown below. Here, the following are the global...