Deconvolutions for images
In the case of images, researchers have been looking for decoding operations acting as the inverse of the encoding convolutions.
The first application was the analysis and understanding of convolutional networks, as seen in Chapter 2, Classifying Handwritten Digits with a Feedforward Network, composed of convolutional layers, max-pooling layers and rectified linear units. To better understand the network, the idea is to visualize the parts of an image that are most discriminative for a given unit of a network: one single neuron in a high level feature map is left non-zero and, from that activation, the signal is retro-propagated back to the 2D input.
To reconstruct the signal through the max pooling layers, the idea is to keep track of the position of the maxima within each pooling region during the forward pass. Such architecture, named DeConvNet can be shown as:
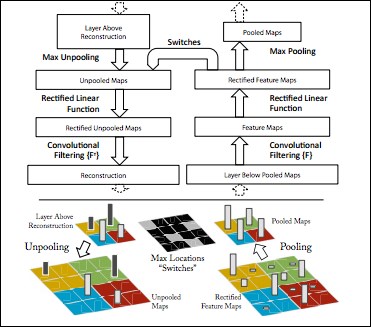
Visualizing and understanding convolutional networks
The signal is retro-propagated to the position that...