Simple recurrent network
An RNN is a network applied at multiple time steps but with a major difference: a connection to the previous state of layers at previous time steps named hidden states :
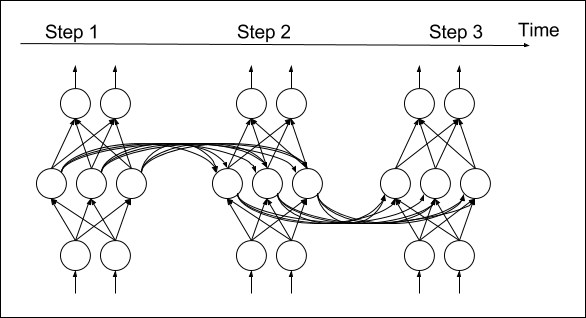
This can be written in the following form:
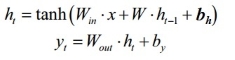
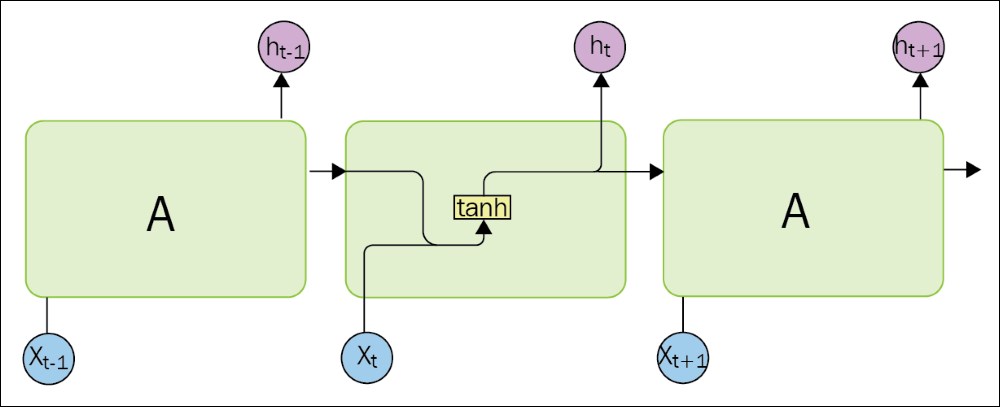
An RNN can be unrolled as a feedforward network applied on the sequence as input and with shared parameters between different time steps.
Input and output's first dimension is time, while next dimensions are for the data dimension inside each step. As seen in the previous chapter, the value at a time step (a word or a character) can be represented either by an index (an integer, 0-dimensional) or a one-hot-encoding vector (1-dimensional). The former representation is more compact in memory. In this case, input and output sequences will be 1-dimensional represented by a vector, with one dimension, the time:
x = T.ivector() y = T.ivector()
The structure of the training program remains the same as in Chapter 2, Classifying Handwritten Digits with a Feedforward...