Performance Metrics
There are different evaluation metrics in machine learning, and these depend on the type of data and the requirements. Some of the metrics are as follows:
- Confusion matrix
- Precision
- Recall
- Accuracy
- F1 score
Confusion Matrix
A confusion matrix is a table that is used to define the performance of the classification model on the test data for which the actual values are known. To understand this better, look at the following figure, showing predicted and actual values:
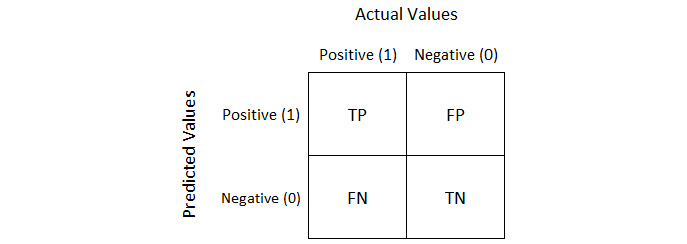
Figure 1.54: Predicted versus actual values
Let's examine the concept of a confusion matrix and its metrics, TP, TN, FP, and FN, in detail. Assume you are building a model that predicts pregnancy:
- TP (True Positive): The sex is female and she is actually pregnant, and your model also predicted True.
- FP (False Positive): The sex is male and your model predicted True, which cannot happen. This is a type of error called a Type 1 error.
- FN (False Negative): The sex is female and she is actually pregnant, and the model predicts False, which is also an error. This is called a Type 2 error.
- TN (True Negative): The sex is male and the prediction is False; that is a True Negative.
The Type 1 error is a more dangerous error than the Type 2 error. Depending on the problem, we have to figure out whether we need to reduce Type 1 errors or Type 2 errors.
Precision
Precision is the ratio of TP outcomes to the total number of positive outcomes predicted by a model. The precision looks at how precise our model is as follows:

Figure 1.55: Precision equation
Recall
Recall calculates what proportion of the TP outcomes our model has predicted:

Figure 1.56: Recall equation
Accuracy
Accuracy calculates the ratio of the number of positive predictions made by a model out of the total number of predictions made:

Figure 1.57: Accuracy equation
F1 score
F1 score is another accuracy measure, but one that allows us to seek a balance between precision and recall:

Figure 1.58: F1-score
When considering the performance of a model, we have to understand two other important concepts of prediction error: bias and variance.
What is bias?
Bias is how far a predicted value is from the actual value. High bias means the model is very simple and is not capable of capturing the data's complexity, causing what's called underfitting.
What is variance?
High variance is when the model performs too well on the trained dataset. This causes overfitting and makes the model too specific to the train data, meaning the model does not perform well on test data.
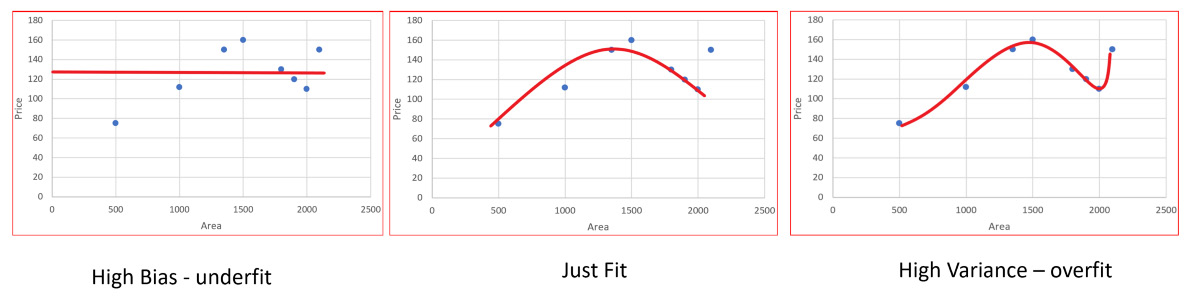
Figure 1.59: High variance
Assume you are building a linear regression model to predict the market price of cars in a country. Let's say you have a large dataset about the cars and their prices, but there are still some more cars whose prices need to be predicted.
When we train our model with the dataset, we want our model to just find that pattern within the dataset, nothing more, because if it goes beyond that, it will start to memorize the train set.
We can improve our model by tuning its hyperparameters - there is more on this later in the book. We work towards minimizing the error and maximizing the accuracy by using another dataset, called the validation set. The first graph shows that the model has not learned enough to predict well in the test set. The third graph shows that the model has memorized the training dataset, which means the accuracy score will be 100, with 0 error. But if we predict on the test data, the middle model will outperform the third.