Neural networks model complex nonlinear functions, such as sin(x), x**2, and x**3, to name a few simple ones and are made of a network (stack) of layers. These layers could be a mixture of convolutional, recurrent, or simply feedforward layers. Each layer is made up of neurons. A neuron has two ingredients to model nonlinearity—the weighted sum from previous layers followed by an activation function. The neural network tries to learn the distribution of given training data in an iterative manner. Once the neural network is built in terms of layers stack by specifying activation functions, an objective function (also known as the loss function) needs to be defined to improve model weights using an appropriate optimizer. There are multiple kinds of loss functions, such as the sum of squares loss used for regression problems and cross-entropy...




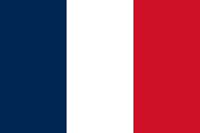


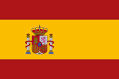




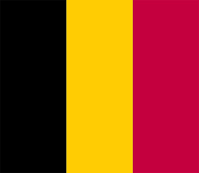
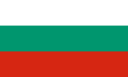
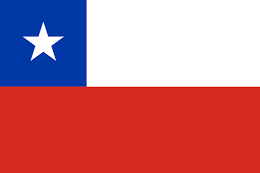
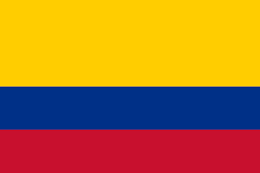
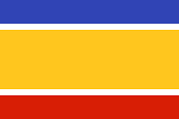

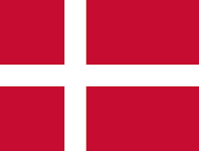



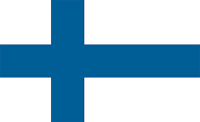

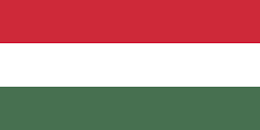






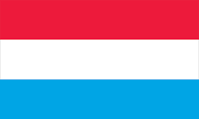
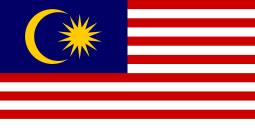

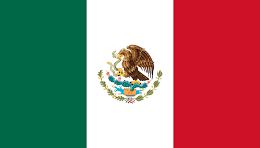

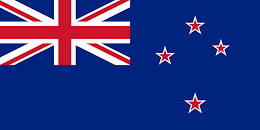







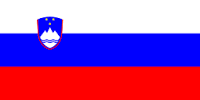




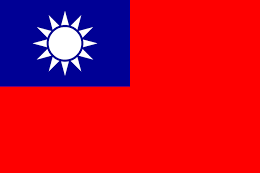


