Machine learning (ML) is about using a set of statistical and mathematical algorithms to perform tasks such as concept learning, predictive modeling, clustering, and mining useful patterns can be performed. The ultimate goal is to improve the learning in such a way that it becomes automatic, so that no more human interactions are needed, or to reduce the level of human interaction as much as possible.
We now refer to a famous definition of ML by Tom M. Mitchell (Machine Learning, Tom Mitchell, McGraw Hill, 1997), where he explained what learning really means from a computer science perspective:
Based on the preceding definition, we can conclude that a computer program or machine can do the following:
- Learn from data and histories
- Be improved with experience
- Interactively enhance a model that can be used to predict an outcome
A typical ML function can be formulated as a convex optimization problem for finding a minimizer of a convex function f that depends on a variable vector w (weights), which has d records. Formally, we can write this as the following optimization problem:
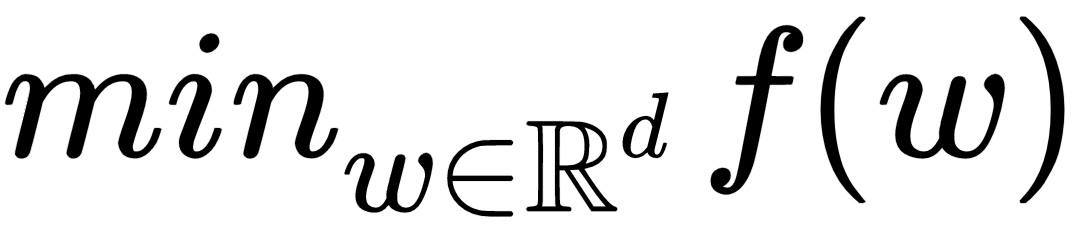
Here, the objective function is of the form:

Here, the vectors are the training data points for 1≤i≤n, and are their corresponding labels that we want to predict eventually. We call the method linear if L(w;x,y) can be expressed as a function of wTx and y.
The objective function f has two components:
- A regularizer that controls the complexity of the model
- The loss that measures the error of the model on the training data
The loss function L(w;) is typically a convex function in w. The fixed regularization parameter λ≥0 defines the trade-off between the two goals of minimizing the loss on the training error and minimizing model complexity to avoid overfitting. Throughout the chapters, we will learn in details on different learning types and algorithms.
On the other hand, deep neural networks (DNN) form the core of deep learning (DL) by providing algorithms to model complex and high-level abstractions in data and can better exploit large-scale datasets to build complex models
There are some widely used deep learning architectures based on artificial neural networks: DNNs, Capsule Networks, Restricted Boltzmann Machines, deep belief networks, factorization machines and recurrent neural networks.
These architectures have been widely used in computer vision, speech recognition, natural language processing, audio recognition, social network filtering, machine translation, bioinformatics and drug design. Throughout the chapters, we will see several real-life examples using these architectures to achieve state-of-the art predictive accuracy.