Reinforcement learning (RL) is a popular and promising branch of artificial intelligence that involves making smarter models and agents that can automatically determine ideal behavior based on changing requirements. Reinforcement Learning Algorithms with Python will help you master RL algorithms and understand their implementation as you build self-learning agents.
Starting with an introduction to the tools, libraries, and setup needed to work in the RL environment, this book covers the building blocks of RL and delves into value-based methods such as the application of Q-learning and SARSA algorithms. You'll learn how to use a combination of Q-learning and neural networks to solve complex problems. Furthermore, you'll study policy gradient methods, TRPO, and PPO, to improve performance and stability, before moving on to the DDPG and TD3 deterministic algorithms. This book also covers how imitation learning techniques work and how Dagger can teach an agent to fly. You'll discover evolutionary strategies and black-box optimization techniques. Finally, you'll get to grips with exploration approaches such as UCB and UCB1 and develop a meta-algorithm called ESBAS.
By the end of the book, you'll have worked with key RL algorithms to overcome challenges in real-world applications, and you'll be part of the RL research community.




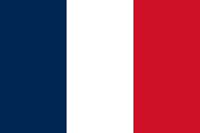


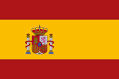




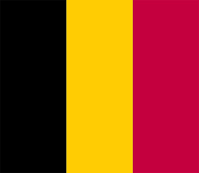
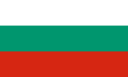
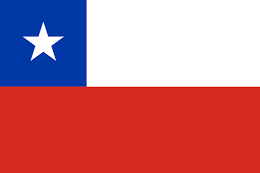
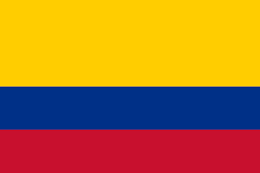
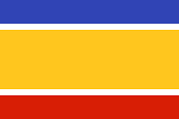

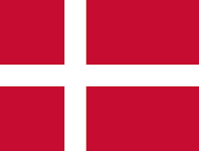



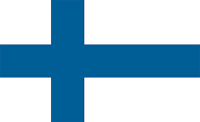

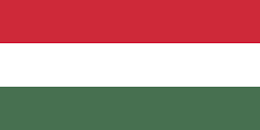






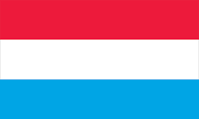
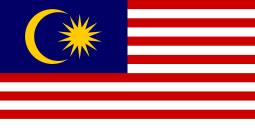

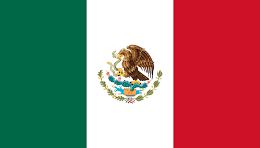

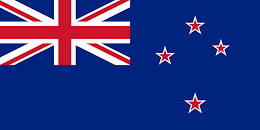







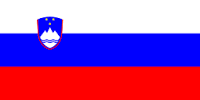




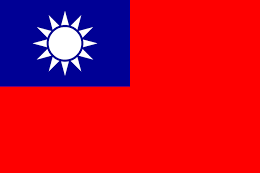


