From raw data to embeddings in vector stores
Embeddings convert any form of data (text, images, or audio) into real numbers. Thus, a document is converted into a vector. These mathematical representations of documents allow us to calculate the distances between documents and retrieve similar data.
The raw data (books, articles, blogs, pictures, or songs) is first collected and cleaned to remove noise. The prepared data is then fed into a model such as OpenAI text-embedding-3-small
, which will embed the data. Activeloop Deep Lake, for example, which we will implement in this chapter, will break a text down into pre-defined chunks defined by a certain number of characters. The size of a chunk could be 1,000 characters, for instance. We can let the system optimize these chunks, as we will implement them in the Optimizing chunking section of the next chapter. These chunks of text make it easier to process large amounts of data and provide more detailed embeddings of a document, as shown here:
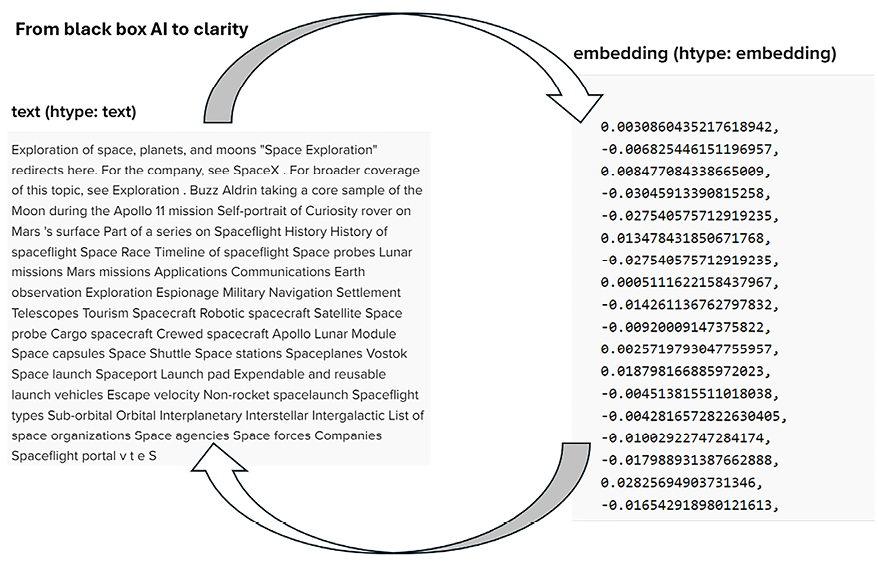
Figure 2.1: Excerpt of an Activeloop vector store dataset record
Transparency has been the holy grail in AI since the beginning of parametric models, in which the information is buried in learned parameters that produce black box systems. RAG is a game changer, as shown in Figure 2.1, because the content is fully traceable:
- Left side (Text): In RAG frameworks, every piece of generated content is traceable back to its source data, ensuring the output’s transparency. The OpenAI generative model will respond, taking the augmented input into account.
- Right side (Embeddings): Data embeddings are directly visible and linked to the text, contrasting with parametric models where data origins are encoded within model parameters.
Once we have our text and embeddings, the next step is to store them efficiently for quick retrieval. This is where vector stores come into play. A vector store is a specialized database designed to handle high-dimensional data like embeddings. We can create datasets on serverless platforms such as Activeloop, as shown in Figure 2.2. We can create and access them in code through an API, as we will do in the Building a RAG pipeline section of this chapter.
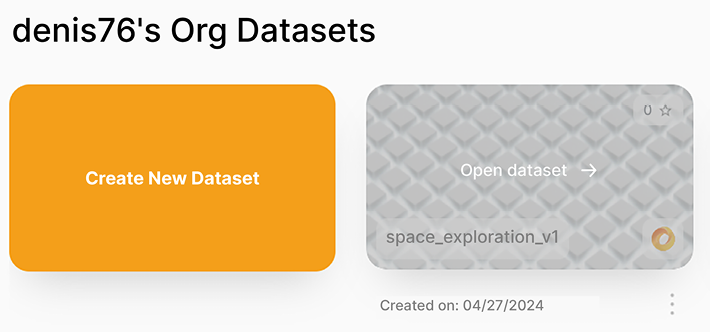
Figure 2.2: Managing datasets with vector stores
Another feature of vector stores is their ability to retrieve data with optimized methods. Vector stores are built with powerful indexing methods, which we will discuss in the next chapter. This retrieving capacity allows a RAG model to quickly find and retrieve the most relevant embeddings during the generation phase, augment user inputs, and increase the model’s ability to produce high-quality output.
We will now see how to organize a RAG pipeline that goes from data collection, processing, and retrieval to augmented-input generation.