Understanding computational graphs and sessions
As we have learned, every computation in TensorFlow is represented by a computational graph. They consist of several nodes and edges, where nodes are mathematical operations, such as addition and multiplication, and edges are tensors. Computational graphs are very efficient at optimizing resources and promote distributed computing.
A computational graph consists of several TensorFlow operations, arranged in a graph of nodes.
A computational graph helps us to understand the network architecture when we work on building a really complex neural network. For instance, let's consider a simple layer, h = Relu(WX + b). Its computational graph would be represented as follows:
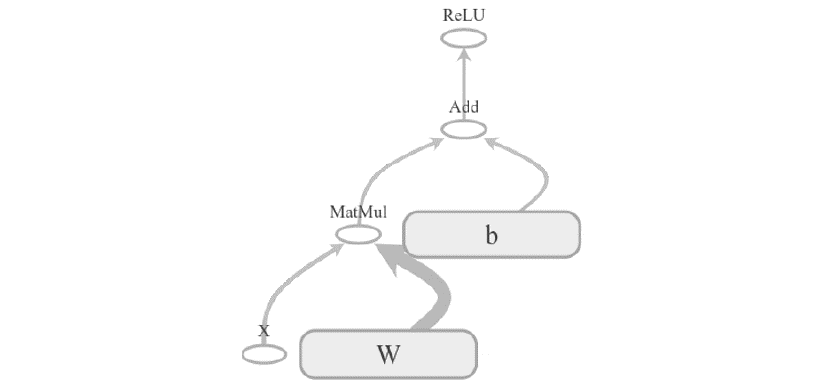
Figure 8.1: Computational graph
There are two types of dependency in the computational graph, called direct and indirect dependency. Say we have node b
, the input of which is dependent on the output of node a
; this type of dependency is called...