



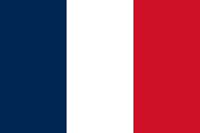


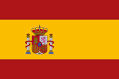



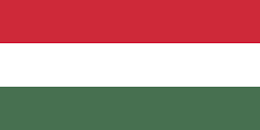

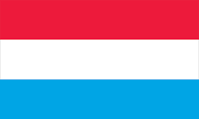





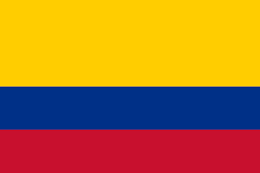
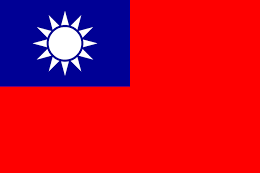
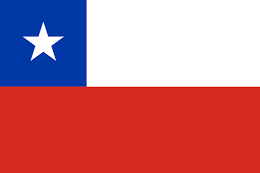



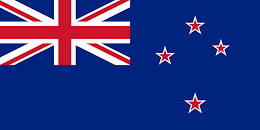
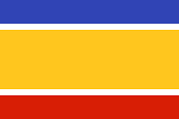
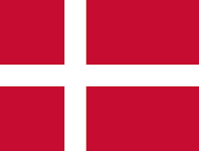
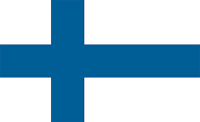







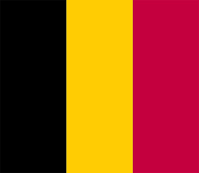

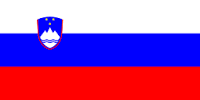





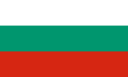


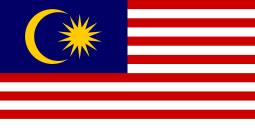



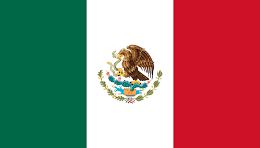

This article is the second part of a series of articles, please refer to Part 2 for learning how to Get to grips with LangChain framework and how to utilize it for building LLM-powered Apps
LangChain is a powerful and open-source Python library specifically designed to enhance the usability, accessibility, and versatility of Large Language Models (LLMs) such as GPT-3 (Generative Pre-trained Transformer 3), BERT(Bidirectional Encoder Representations from Transformers), BLOOM (BigScience Large Open-science Open-access Multilingual Language Model). It provides developers with a comprehensive set of tools to seamlessly combine multiple prompts, creating a harmonious orchestra for working with LLMs effortlessly. The project was initiated by Harrison Chase, with the first commit made in late October 2022. In just a few weeks, LangChain gained immense popularity within the open-source community.
Image 1: The popularity of the LangChain Python library
To fully grasp the fundamentals of LangChain and utilize it effectively — understanding the fundamentals of LLMs is essential. In simple terms, LLMs are sophisticated language models or AI systems that have been extensively trained on massive amounts of text data to comprehend and generate human-like language. Albeit their powerful capabilities, LLMs are generic in nature i.e. lacking domain-specific knowledge or expertise. For instance, when addressing queries in fields like medicine or law, while an LLM can provide general insights, it, however, may struggle to offer in-depth or nuanced responses that require specialized expertise. Alongside such limitations, LLMs are susceptible to biases and inaccuracies present in training data which can yield contextually plausible, yet incorrect outputs. This is where LangChain shines — serving as an open-source library that leverages the power of LLMs and mitigates their drawbacks by providing abstractions and a diverse range of modules, akin to Lego blocks, thus facilitating intuitive integration with other tools and knowledge bases.
In brief, LangChain presents a useful approach for handling text data, wherein the initial step involves preprocessing of the large corpus by segmenting it into smaller chunks or summaries. These chunks are then transformed into vector representations, enabling efficient comparisons and retrieval of similar chunks when questions are posed. This approach of preprocessing, real-time data collection, and interaction with the LLM is not only applicable to the specific context but can also be effectively utilized in other scenarios like code and semantic search.
Image 2 - Typical workflow of Langchain ( Image created by Author)
A typical workflow of LangChain involves several steps that enable efficient interaction between the user, the preprocessed text corpus, and the LLM.
Notably, the strengths of LangChain lie in its provision of an abstraction layer, streamlining the intricate process of composing and integrating these text components, thereby enhancing overall efficiency and effectiveness.
The core concept behind LangChain is its ability to connect a “Chain of thoughts” around LLMs, as evident from its name. However, LangChain is not limited to just a few LLMs — it provides a wide range of components that work together as building blocks for advanced use cases involving LLMs. Now, let’s delve into the various components that the LangChain library offers, making our work with LLMs easier and more efficient.
Image 3: LangChain features at a glance. (Image created by Author)
Overall, LangChain provides a broad spectrum of features and modules that greatly enhance our interaction with LLMs. In the subsequent sections, we will explore the practical usage of LangChain and demonstrate how to build simple demo web applications using its capabilities.
Avratanu Biswas, Ph.D. Student ( Biophysics ), Educator, and Content Creator, ( Data Science, ML & AI ).