Firstly, temporal difference (TD) is the difference of the value estimates between two time steps. It is different from the outcome-based Monte Carlo approach where a full look ahead till the end of the episode is done in order to update the learning parameters. In case of temporal difference learning, only one step look ahead is done and a value estimate of the state at the next step is used to update the current state's value estimate. Thus, learning parameters update along the way. Different rules to approach temporal difference learning are the TD(1), TD(0), and TD() rules. The basic notion in all the approaches is that the value estimate of the next step is used to update the current state's value estimate.




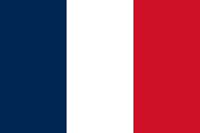


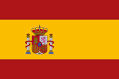




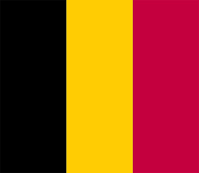
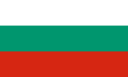
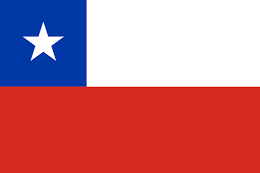
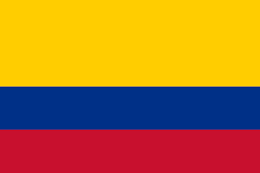
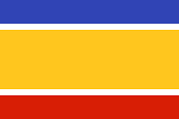

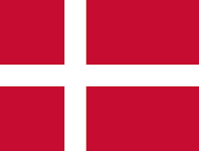



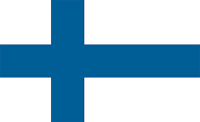

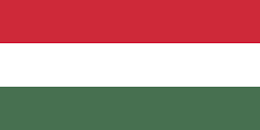






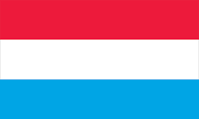
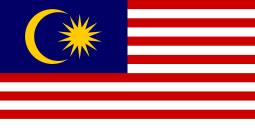

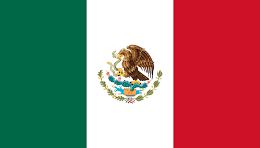

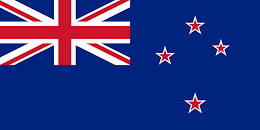







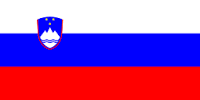




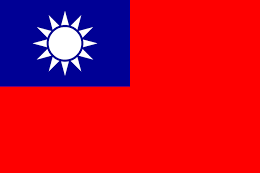


