Deep deterministic policy gradient
In Chapter 8, Atari Games with Deep Q Network, we looked at how DQN works and we applied DQNs to play Atari games. However, those are discrete environments where we have a finite set of actions. Think of a continuous environment space like training a robot to walk; in those environments it is not feasible to apply Q learning because finding a greedy policy will require a lot of optimization at each and every step. Even if we make this continuous environment discrete, we might lose important features and end up with a huge set of action spaces. It is difficult to attain convergence when we have a huge action space.
So we use a new architecture called Actor Critic with two networks—Actor and Critic. The Actor Critic architecture combines the policy gradient and state action value functions. The role of the Actor network is to determine the best actions in the state by tuning the parameterÂ
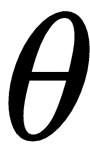
, and the role of the Critic is to evaluate the action produced by the...