Data normalization is used when you want to adjust the values in the feature vector so that they can be measured on a common scale. One of the most common forms of normalization that is used in machine learning adjusts the values of a feature vector so that they sum up to 1.
Normalization
Getting ready
To normalize data, the preprocessing.normalize() function can be used. This function scales input vectors individually to a unit norm (vector length). Three types of norms are provided, l1, l2, or max, and they are explained next. If x is the vector of covariates of length n, the normalized vector is y=x/z, where z is defined as follows:
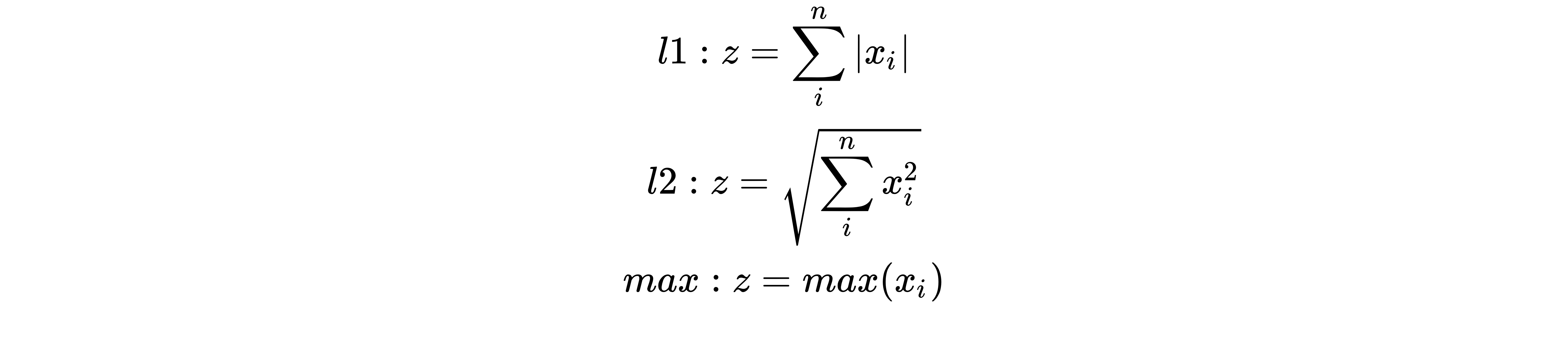
The norm is a function that assigns a positive length to each vector belonging to a vector space, except 0.
How to do it...
Let's see how to normalize data in Python:
- As we said, to normalize data, the preprocessing.normalize() function can be used as follows (we will use the same data as in the previous recipe):
>> data_normalized = preprocessing.normalize(data, norm='l1', axis=0)
- To display the normalized array, we will use the following code:
>> print(data_normalized)
The following output is returned:
[[ 0.75 -0.17045455 0.47619048 -0.45762712]
[ 0. 0.45454545 -0.07142857 0.1779661 ]
[ 0.25 0.375 -0.45238095 -0.36440678]]
This is used a lot to make sure that datasets don't get boosted artificially due to the fundamental nature of their features.
- As already mentioned, the normalized array along the columns (features) must return a sum equal to 1. Let's check this for each column:
>> data_norm_abs = np.abs(data_normalized)
>> print(data_norm_abs.sum(axis=0))
In the first line of code, we used the np.abs() function to evaluate the absolute value of each element in the array. In the second row of code, we used the sum() function to calculate the sum of each column (axis=0). The following results are returned:
[1. 1. 1. 1.]
Therefore, the sum of the absolute value of the elements of each column is equal to 1, so the data is normalized.
How it works...
In this recipe, we normalized the data at our disposal to the unitary norm. Each sample with at least one non-zero component was rescaled independently of other samples so that its norm was equal to one.
There's more...
Scaling inputs to a unit norm is a very common task in text classification and clustering problems.
See also
- Scikit-learn's official documentation of the sklearn.preprocessing.normalize() function: https://scikit-learn.org/stable/modules/generated/sklearn.preprocessing.normalize.html.