Lag plots
A lag plot is a scatter plot for a time series and the same data lagged. With such a plot, we can check whether there is a possible correlation between CPU transistor counts this year and the counts of the previous year, for instance. The lag_plot()
 Pandas function in pandas.tools.plotting
can draw a lag plot. Draw a lag plot with the default lag of 1
for the CPU transistor counts, as follows:
lag_plot(np.log(df['trans_count']))
Refer to the following plot for the end result:
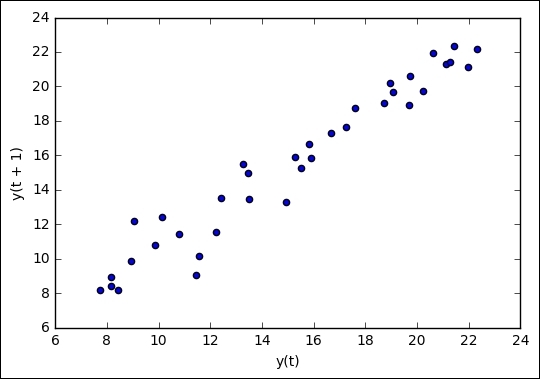
The following code for the lag plot example can also be found in the ch-06.ipynb
file in this book's code bundle:
import matplotlib.pyplot as plt import numpy as np import pandas as pd from pandas.tools.plotting import lag_plot df = pd.read_csv('transcount.csv') df = df.groupby('year').aggregate(np.mean) gpu = pd.read_csv('gpu_transcount.csv') gpu = gpu.groupby('year').aggregate(np.mean) df = pd.merge(df, gpu, how='outer', left_index...