Model-agnostic meta-learning (MAML) attempts to solve the shortcomings of the gradient-descent approach by providing better weight initialization for every new task. The key idea of this approach is to train the models' parameters using a different dataset. When using it for a new task, the model gives better performance by using already initialized parameters to fine-tune the architecture through one or more gradient steps. This method of training a model's parameters so that a few gradient steps can optimize the loss function can also be viewed, from a feature-learning standpoint, as building an internal representation. In this approach, we choose a generic model's architecture so that it can be used for various tasks. The primary contribution of MAML is a simple model- and task-agnostic fast learning algorithm.
...



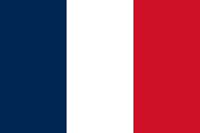


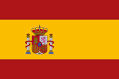




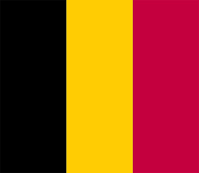
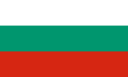
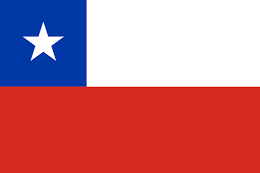
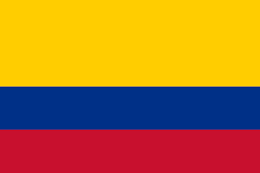
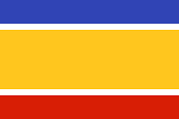

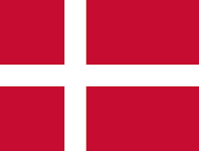



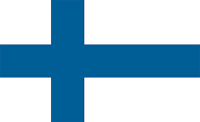

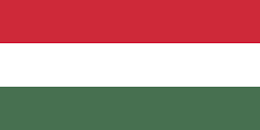






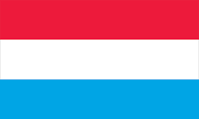
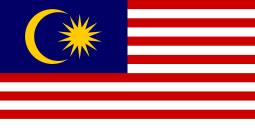

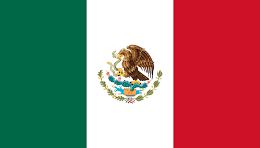

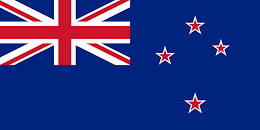







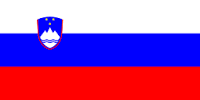




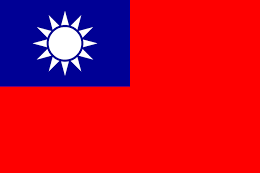


