Multiple linear regression
Sometimes it makes more sense to include more predictors (that is, the independent variables) to find the value of the dependent variable (that is, the predicted variable). For example, predicting the price of a house based only on the total area is probably not a good idea. Maybe the price also depends on the number of bathrooms and the distance from several required facilities, such as schools, grocery stores, and so on.
So we might have a dataset as shown next, and the problem we pose for our linear regression model is to predict the price of a new house given all the other parameters:
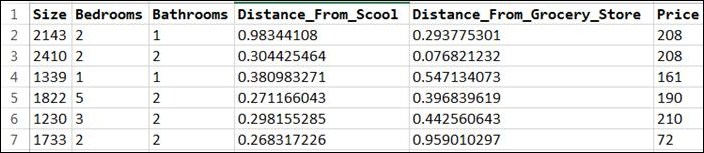
In this case, the model can be represented as a linear combination of all the predictors, as follows:

Here, the theta values represent the parameters we must select to fit the model. In vectorized form, this can be written as:
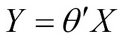
Theta can be calculated by the following formula:
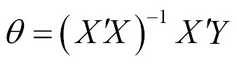
So using the MathNet.Fsharp
package, this can be calculated as follows:
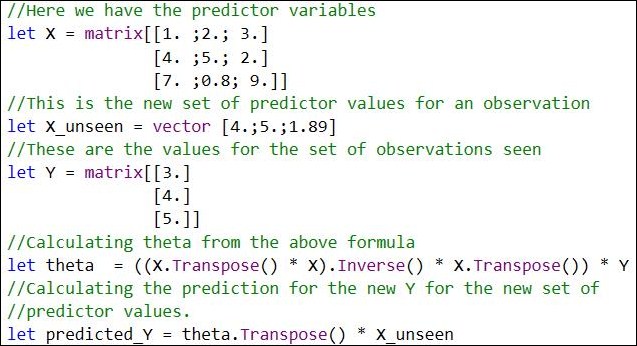
Previously, in Chapter 1, Introduction...