In this chapter, we have taken a conceptual walk through some of the most common methods used to compute the posterior distribution, including variational methods and Markov Chain Monte Carlo methods. We have put special emphasis on universal inference engines, methods that are designed to work on any given model (or at least a broad range of models). These methods are the core of any probabilistic programming language as they allow for automatic inference, letting users concentrate on iterative model design and interpretations of the results. We also discussed numerical and visual tests for diagnosing samples. Without good approximations to the posterior distribution, all the advantages and flexibility of the Bayesian framework vanish, so evaluating the quality of the inference process is crucial for us to be confident of the quality of the inference process itself.
...



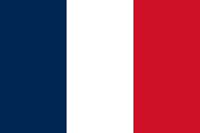


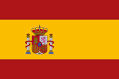



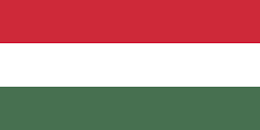

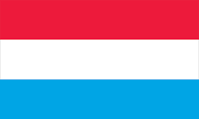





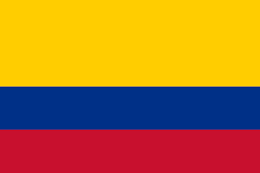
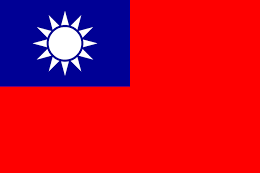
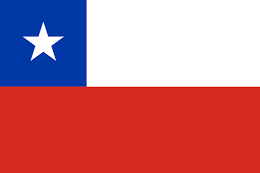



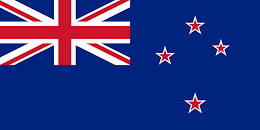
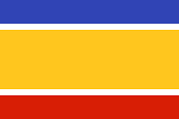
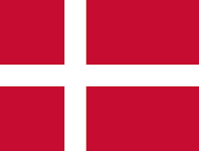
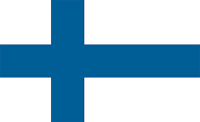







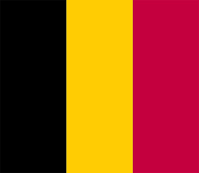

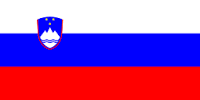





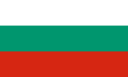


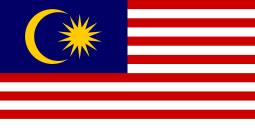



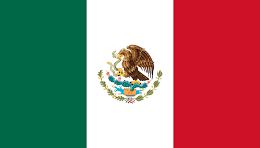
