




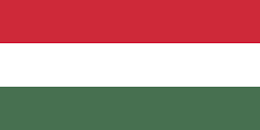

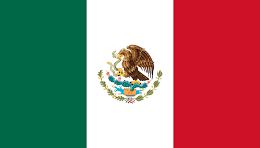


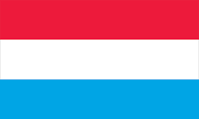



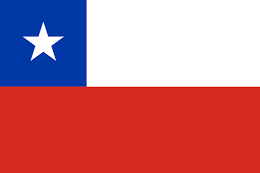



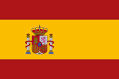


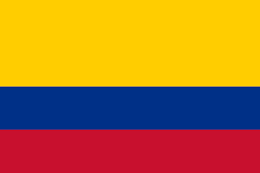
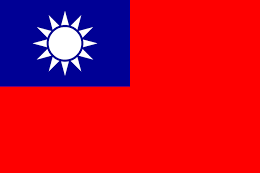


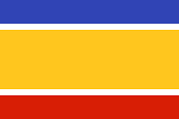
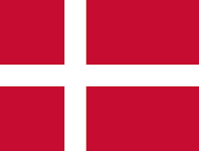
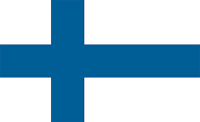



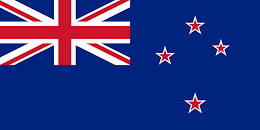


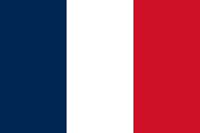



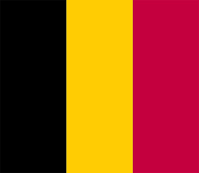

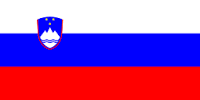




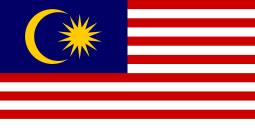


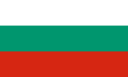




Dive deeper into the world of AI innovation and stay ahead of the AI curve! Subscribe to our AI_Distilled newsletter for the latest insights. Don't miss out – sign up today!
The process of detecting and extracting emotion from text is referred to as sentiment analysis. It's a powerful tool that can help understand the views of consumers, look at brand ratings, and find satisfaction with customers. Genetic AI models like GPT 3, PaLM, and Bard can change the way we think about sentiment analysis. It is possible to train these models with the aim of understanding human language's nuances, and detecting sentiment in any complicated or subtle text.
Benefits of using generative AI for sentiment analysis
There are several benefits to using generative AI for sentiment analysis, including:
There are two main ways to use generative AI for sentiment analysis:
In this example, we will use the PaLM API to perform sentiment analysis on a customer review.
Input:
import nltk
from nltk.sentiment.vader import SentimentIntensityAnalyzer
# Download the VADER lexicon for sentiment analysis (run this once)
nltk.download('vader_lexicon')
def analyze_sentiment(sentence):
# Initialize the VADER sentiment intensity analyzer
analyzer = SentimentIntensityAnalyzer()
# Analyze the sentiment of the sentence
sentiment_scores = analyzer.polarity_scores(sentence)
# Determine the sentiment based on the compound score
if sentiment_scores['compound'] >= 0.05:
return 'positive'
elif sentiment_scores['compound'] <= -0.05:
return 'negative'
else:
return 'neutral'
# Example usage with a positive sentence
positive_sentence = "I am thrilled with the results! The team did an amazing job!"
sentiment = analyze_sentiment(positive_sentence)
print(f"Sentiment: {sentiment}")
Output:
Sentiment: positive
In order to analyze the emotion in a particular sentence we have created a function that divides it into categories based on its sentiment score and labels it as positive, unfavorable, or neutral. For example, a positive sentence is analyzed and the results show a "positive" sentiment.
Input:
import nltk
from nltk.sentiment.vader import SentimentIntensityAnalyzer
# Download the VADER lexicon for sentiment analysis (run this once)
nltk.download('vader_lexicon')
def analyze_sentiment(sentence):
# Initialize the VADER sentiment intensity analyzer
analyzer = SentimentIntensityAnalyzer()
# Analyze the sentiment of the sentence
sentiment_scores = analyzer.polarity_scores(sentence)
# Determine the sentiment based on the compound score
if sentiment_scores['compound'] >= 0.05:
return 'positive'
elif sentiment_scores['compound'] <= -0.05:
return 'negative'
else:
return 'neutral'
# Example usage with a negative sentence
negative_sentence = "I am very disappointed with the service. The product didn't meet my expectations."
sentiment = analyze_sentiment(negative_sentence)
print(f"Sentiment: {sentiment}")
Output:
Sentiment: negative
We have set up a function to evaluate the opinions of some sentences and then classify them according to their opinion score, which we refer to as Positive, Negative, or Neutral. To illustrate this point, an analysis of the negative sentence is carried out and the output indicates a "negative" sentiment.
Input:
import nltk
from nltk.sentiment.vader import SentimentIntensityAnalyzer
# Download the VADER lexicon for sentiment analysis (run this once)
nltk.download('vader_lexicon')
def analyze_sentiment(sentence):
# Initialize the VADER sentiment intensity analyzer
analyzer = SentimentIntensityAnalyzer()
# Analyze the sentiment of the sentence
sentiment_scores = analyzer.polarity_scores(sentence)
# Determine the sentiment based on the compound score
if sentiment_scores['compound'] >= 0.05:
return 'positive'
elif sentiment_scores['compound'] <= -0.05:
return 'negative'
else:
return 'neutral'
# Example usage
sentence = "This is a neutral sentence without any strong sentiment."
sentiment = analyze_sentiment(sentence)
print(f"Sentiment: {sentiment}")
Output:
Sentiment: neutral
For every text item, whether it's a customer review, social media post or news report, the PaLM API can be used for sentiment analysis. To do this, select a prompt that tells your model what it wants to be doing and then request API by typing in the following sentence as positive, negative, or neutral. A prediction that you can print to the console or use in your application will then be generated by this model.
Sentiment analysis with generative AI can be used in a wide variety of applications, including:
While generative AI has the potential to revolutionize sentiment analysis, there are also some challenges that need to be addressed:
The potential for Generative AI to disrupt sentiment analysis is enormous. The generative AI models can achieve very high accuracy, scale to analyze a large volume of text, and be custom designed in order to meet the specific needs of different companies and organizations. In order to analyze sentiment for a broad range of text data, generative AI models can be used if you use fast engineering and quality tuning.
A powerful new tool that can be applied to understand and analyze human language in new ways is sentiment analysis with generative artificial intelligence. As models of generative AI are improving, we can expect that this technology will be applied to a wider variety of applications and has an important impact on our daily lives and work.
Sangita Mahala is a passionate IT professional with an outstanding track record, having an impressive array of certifications, including 12x Microsoft, 11x GCP, 2x Oracle, and LinkedIn Marketing Insider Certified. She is a Google Crowdsource Influencer and IBM champion learner gold. She also possesses extensive experience as a technical content writer and accomplished book blogger. She is always Committed to staying with emerging trends and technologies in the IT sector.