



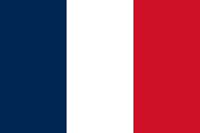


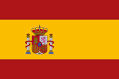



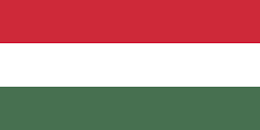

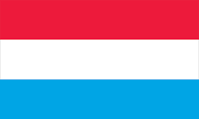





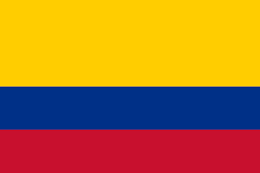
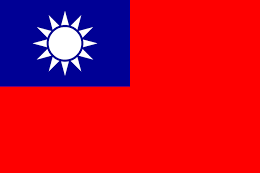
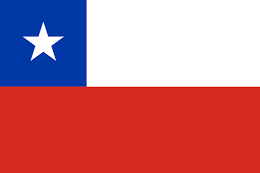



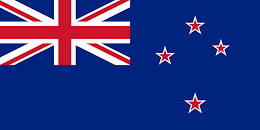
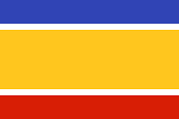
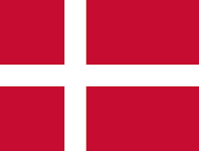
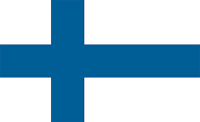







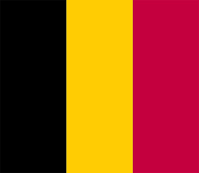

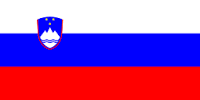





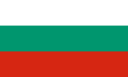


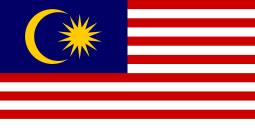



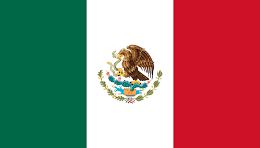

[box type="note" align="" class="" width=""]Our article is an excerpt taken from the book R Data Mining, written by Andrea Cirillo. If you are a budding data scientist or a data analyst with basic knowledge of R, and you want to get into the intricacies of data mining in a practical manner, be sure to check out this book.[/box]
In today’s post, we will analyze R's strengths, and understand why it is a savvy idea to learn this programming language for data mining.
You know that R is really popular, but why? R is not the only data analysis language out there, and neither is it the oldest one; so why is it so popular?
If looking at the root causes of R's popularity, we definitely have to mention these three:
One of the main reasons the adoption of R is spreading is its open source nature. R binary code is available for everyone to download, modify, and share back again (only in an open source way). Technically, R is released with a GNU general public license, meaning that you can take it and use it for whatever purpose; but you have to share every derivative with a GNU general public license as well.
These attributes fit well for almost every target user of a statistical analysis language:
You could imagine the R language as an expandable board game. You know, games like 7 Wonders or Carcassonne, with a base set of characters and places and further optional places and characters, increasing the choices at your disposal and maximizing the fun. The R language can be compared to this kind of game.
There is a base version of R, containing a group of default packages that are delivered along with the standard version of the software (you can skip to the Installing R and writing R code section for more on how to obtain and install it). The functionalities available through the base version are mainly related to file system manipulation, statistical analysis, and data Visualization.
While this base version is regularly maintained and updated by the R core team, virtually every R user can add further new functionalities to those available within the package, developing and sharing custom packages.
This is basically how the package development and sharing flow works:
install.packages("ggplot2")
library(ggplot2)
As you can see, this is a really convenient and effective way to expand R functionalities, and you will soon see how wide the range of functionalities added through additional packages developed by R users is.
More than 9,000 packages are available on CRAN, and this number is sure to increase further, making more and more additional features available to the R community.
As a discipline data visualization encompasses all of the principles and techniques employable to effectively display the information and messages contained within a set of data.
Since we are living in an information-heavy age, the ability to effectively and concisely communicate articulated and complex messages through data visualization is a core asset for any professional. This is exactly why R is experiencing a great response in academic and professional fields: the data visualization capabilities of R place it at the cutting edge of these fields.
R has been noticed for its amazing data visualization features right from its beginning; when some of its peers still showed x axes-built aggregating + signs, R was already able to produce astonishing 3D plots. Nevertheless, a major improvement of R as a data visualization technique came when Auckland's Hadley Wickham developed the highly famous ggplot2 package based on The Grammar of Graphics, introducing into the R world an organic framework for data visualization tasks:
This package alone introduced the R community to a highly flexible way of producing and visualizing almost every kind of data visualization, having also been designed as an expandable tool, in order to add the possibility of incorporating new data visualization techniques as soon as they emerge. Finally, ggplot2 gives you the ability to highly customize your plot, adding every kind of graphical or textual annotation to it.
Nowadays, R is being used by the biggest tech companies, such as Facebook and Google, and by widely circulated publications such as the Economist and the New York Times to visualize their data and convey their information to their stakeholders and readers.
To sum all this up—should you invest your precious time learning R? If you are a professional or a student who could gain advantages from knowing effective and cutting-edge techniques to manipulate, model, and present data, I can only give you a positive opinion: yes. You should definitely learn R, and consider it a long-term investment, since the points of strength we have seen place it in a great position to further expand its influence in the coming years in every industry and academic field.
Now that we are aware of R’s popularity we need to engage with the community to take advantage of it. We will look at alternative and non-exclusive ways of engaging with the community:
Employing community-driven learning material: There are two main kinds of R learning materials developed by the community:
Papers, manuals, and books: The first one is for sure the more traditional one, but you shouldn't neglect it, since those kinds of learning materials are always able to give you a more organic and systematic understanding of the topics they treat. You can find a lot of free material online in the form of papers, manuals, and books.
Let me point out to you the more useful ones:
Online interactive courses: This is probably the most common learning material nowadays. You can find different platforms delivering good content on the R language, the most famous of which are probably DataCamp, Udemy, and Packt itself. What all of them share is a practical and interactive approach that lets you learn the topic directly, applying it through exercises rather than passively looking at someone explaining theoretical stuff.
Asking for help from the community: As soon as you start writing your first lines of R code, and perhaps before you even actually start writing it, you will come up with some questions related to your work. The best thing you can do when this happens is to resort to the community to solve those questions. You will probably not be the first one to come up with that question, and you should therefore first of all look online for previous answers to your question.
Where should you look for answers? You can look everywhere, but most of the time you will find the answer you are looking for on one of the following (listed by the probability of finding the answer there):
I wouldn't suggest you look for answers on Twitter, G+, and similar networks, since they were not conceived to handle these kinds of processes and you will expose yourself to the peril of reading answers that are out of date, or simply incorrect, because no review system is considered.
If it is the case that you are asking an innovative question never previously asked by anyone, first of all, congratulations! That said, in that happy circumstance, you can ask your question in the same places that you previously looked for answers.
Staying ahead of language developments: The R language landscape is constantly changing, thanks to the contributions of many enthusiastic users who take it a step further every day. How can you stay ahead of those changes? This is where social networks come in handy. Following the #rstats hashtag on Twitter, Google+ groups, and similar places, will give you the pulse of the language. Moreover, you will find the R-bloggers aggregator, which delivers a daily newsletter comprised of the R-related blog posts that were published the previous day really useful. Finally, annual R conferences and similar occasions constitute a great opportunity to get in touch with the most notorious R experts, gaining from them useful insights and inspiring speeches about the future of the language.
To summarize, we looked why to choose R as your programming language for data mining and how we can engage with the R community.
If you think this post is useful, you may further check out this book R Data Mining, to leverage data mining techniques across many different industries, including finance, medicine, scientific research, and more.