Training a boosting model with XGBoost
Let’s now see another application of decision trees: boosting. While bagging (used in Random Forest models) is training several trees in parallel, boosting is about training trees sequentially. In this recipe, we will have a quick review of what is boosting, and then train a boosting model with XGBoost, a widely used boosting library.
Getting ready
Let’s have a look at introducing limits of bagging, then see how boosting may address some of those limits and how. Finally, let’s train a model on the already prepared Titanic dataset with XGBoost.
Limits of bagging
Let’s assume we have a binary classification task, and we trained Random Forest in three decision trees on two features. Bagging is expected to perform well if anywhere in the feature space, at least two out of three decision trees are right, as in Figure 4.18.
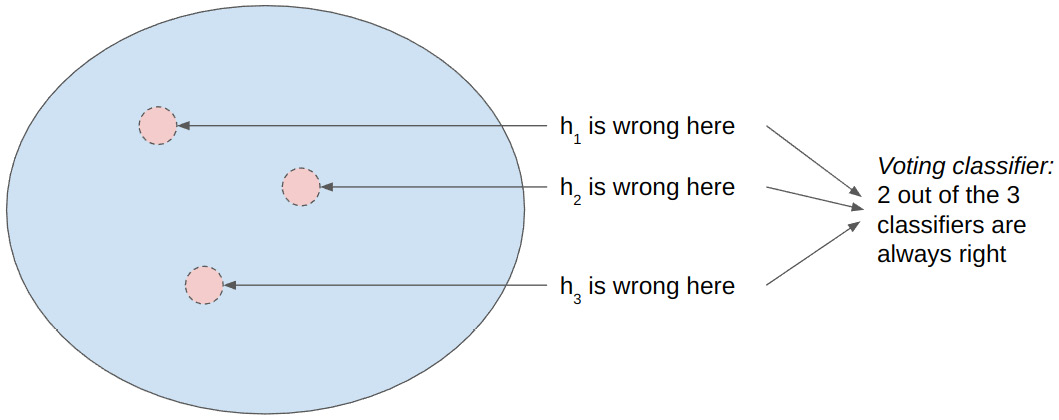
Figure 4.18 – The absence of overlap in dashed circle...