RNNs for modeling sequences
In this section, now that we understand sequences, we can look at the foundations of RNNs. We'll start by introducing the typical structure of an RNN, and we'll see how the data flows through it with one or more hidden layers. We'll then examine how the neuron activations are computed in a typical RNN. This will create a context for us to discuss the common challenges in training RNNs, and explore the modern solution to these challenges—LSTM.
Understanding the structure and flow of an RNN
Let's start by introducing the architecture of an RNN. The following figure shows a standard feedforward neural network and an RNN, in a side by side for comparison:
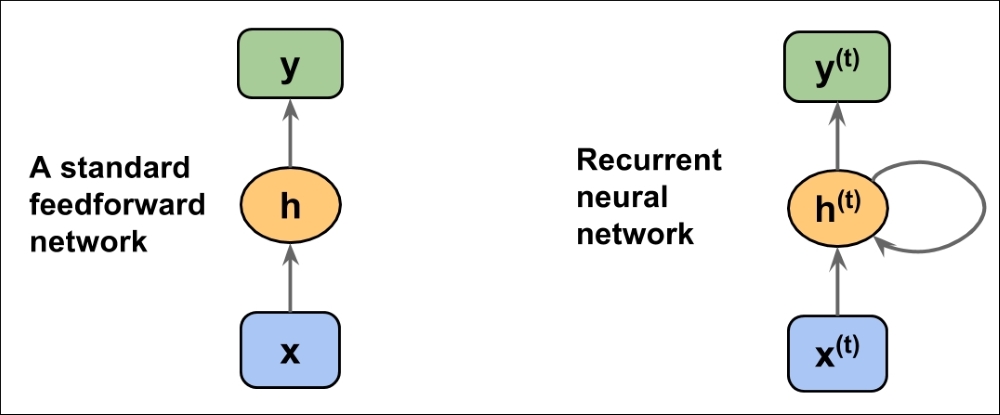
Both of these networks have only one hidden layer. In this representation, the units are not displayed, but we assume that the input layer (x), hidden layer (h), and output layer (y) are vectors which contain many units.
Note
This generic RNN architecture could correspond to the two sequence...