Setting a similarity using the Jaccard index
Quite often, we have to work with sets of data in machine learning. Users like posts, buy products, listen to music, or watch movies. In this case, data is structured in the two columns: 'user
and 'item
.
In order to calculate correlations, we need to work with sets. The Jaccard similarity coefficient is a statistic that measures the similarity between sets. The level of similarity is the calculation of the size of the intersection divided by the size of the union of the sample sets, as shown.
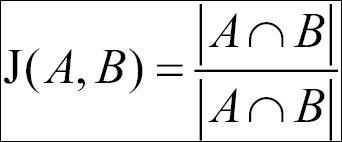
For example, if two users in the dataset are related to the same two items, and each user is also related to a distinct item, the Jaccard similarity indicates the following:
The similarity between item1 and item2 is 100 percent
The similarity between the common and distinct items is 50 percent
The similarity between two distinct items is 0 percent
To begin the implementation, we first need to calculate the item popularity, and then add the popularity back to the...