Now that we have introduced this wonderful architectural pattern, let's take a closer look at it before delving into the possible analytic use cases that can be implemented with this new pattern.
We all know that, at base level, Hadoop gives me vast storage, and has HDFS and a very robust processing engine in the form of MapReduce, which can handle a humongous amount of data and can perform myriad computations. However, it has a long turnaround time (TAT) and it's a batch system that helps us cope with the volume aspect of big data. If we need speed and velocity for processing and are looking for a low–latency solution, we have to resort to a real–time processing engine that could quickly process the latest or the recent data and derive quick insights that are actionable in the current time frame. But along with velocity and quick TAT, we also need newer data to be progressively integrated into the batch system for deep batch analytics that are required to execute on entire data sets. So, essentially we land in a situation where I need both batch and real–time systems, the optimal architectural combination of this pattern is called Lambda architecture (λ).
The following figure captures the high–level design of this pattern:
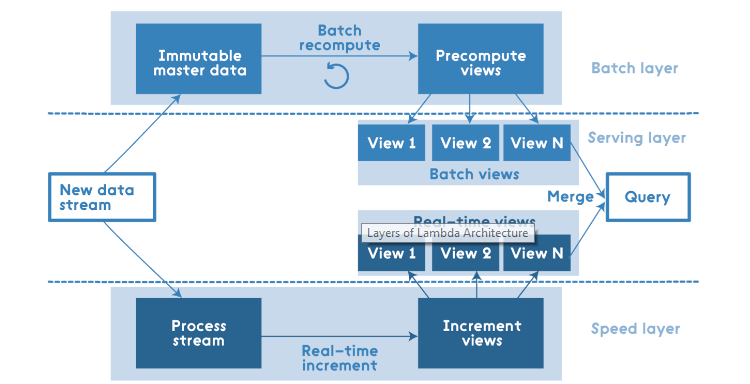
The solution is both technology and language agnostic; at a high–level it has the following three layers:
- The batch layer
- The speed layer
- The serving layer
The input data is fed to both the batch and speed layers, where the batch layer works at creating the precomputed views of the entire immutable master data. This layer is predominately an immutable data store with write once and many bulk reads.
The speed layer handles the recent data and maintains only incremental views over the recent set of the data. This layer has both random reads and writes in terms of data accessibility.
The crux of the puzzle lies in the intelligence of the serving layer, where the data from both the batch and speed layers is merged and the queries are catered for, so we get the best of both the worlds seamlessly. The close to real–time requests are handled from the data from the incremental views (they have low retention policy) from the speed layer while the queries referencing the older data are catered to by the master data views generated in the batch layer. This layer caters only to random reads and no random writes, though it does handle batch computations in the form of queries and joins and bulk writes.
However, Lambda architecture is not a one-stop solution for all hybrid use cases; there are some key aspects that need to be taken care of:
- Always think distributed
- Account and plan for failures
- Rule of thumb: data is immutable
- Finally, plan for failures
Now that we have acquainted ourselves well with the prevalent architectural patterns in real–time analytics, let us talk about the use cases that are possible in this segment:

The preceding figure highlights the high–level domains and various key use cases that may be executed.