Believe it or not, how well a machine learning system can learn is mainly determined by the quality of the training data. Although every learning algorithm has its strengths and weaknesses, differences in performance often come down to the way the data is prepared or represented. Feature engineering can hence be understood as a tool for data representation. Machine learning algorithms try to learn a solution to a problem from sample data, and feature engineering asks: what is the best representation of the sample data to use for learning a solution to the problem?
Remember, a couple of chapters ago, we talked about the whole machine learning pipeline. There, we already mentioned feature extraction, but we didn't really discuss what it is all about. Let's see how it fits in the pipeline:
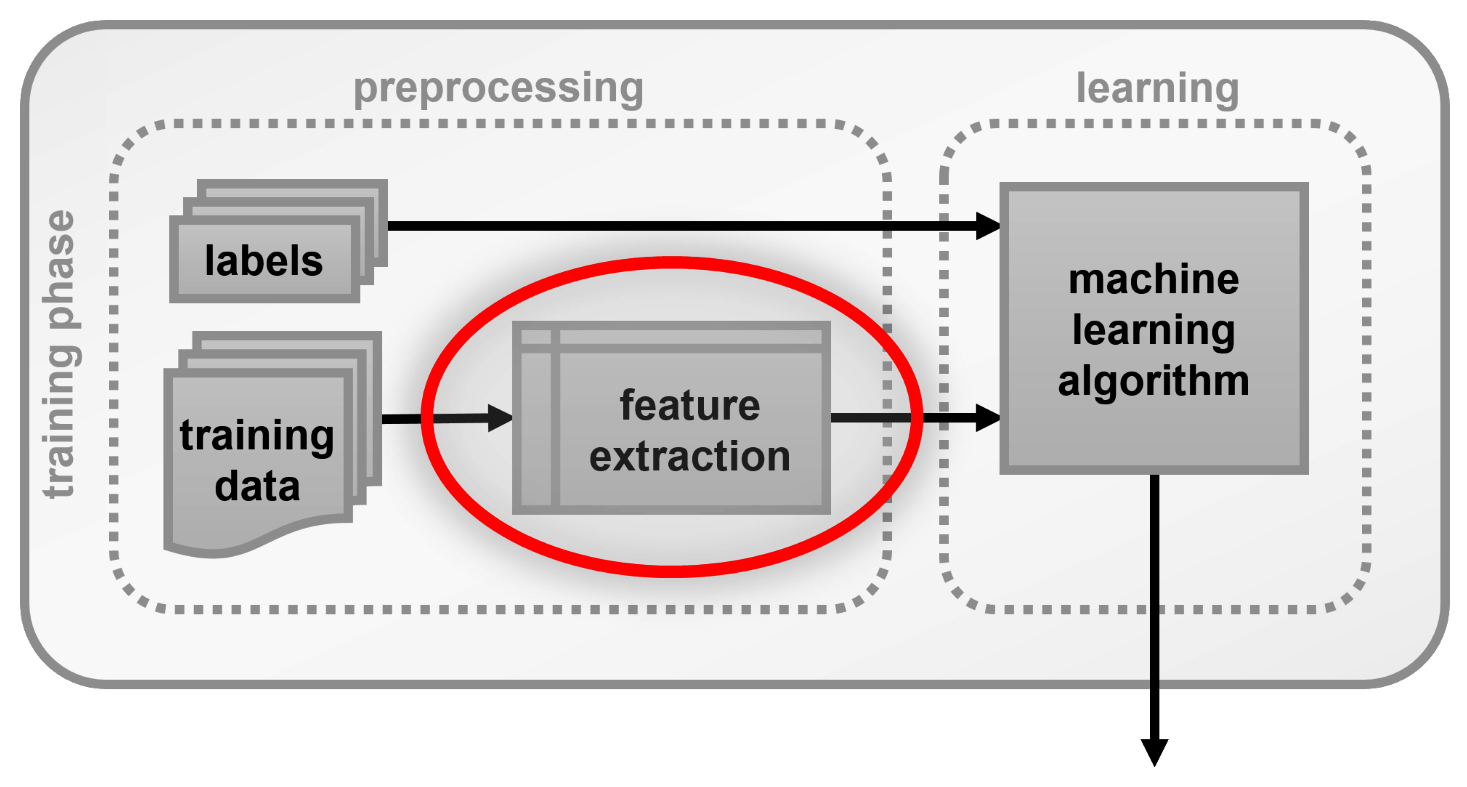
As a brief reminder, we have already discussed...