The concept of one-class support vector machines (SVMs) has been proposed by Schölkopf B, Platt J C, Shawe-Taylor J C, Smola A J, and Williamson R C, in the article Estimating the Support of a High-Dimensional Distribution, Neural Computation, 13/7, 2001 as a method to classify the novelties either as samples drawn from the true data-generating process or as outliers. Let's start with the goal we want to achieve: finding an unsupervised model that, given a sample xi, can yield a binary output yi (conventionally, SVMs outcomes are bipolar: -1 and +1), so that, if xi is inlier yi = +1 and, conversely, yi = -1 if xi is an outlier (more correctly, the authors, in the aforementioned paper, assume that the outcome is 1 for the majority of inliers, which constitute the training set). At a first glance, it can seem a classical supervised problem...




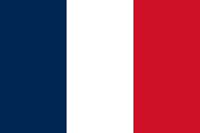


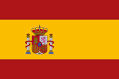



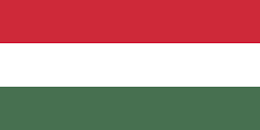

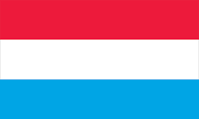





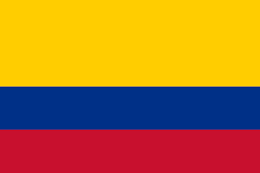
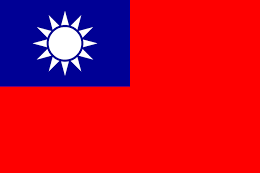
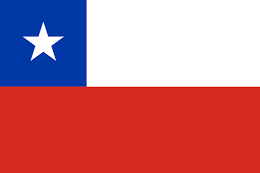



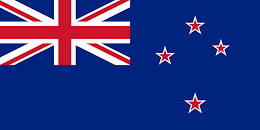
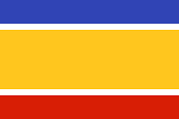
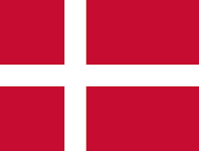
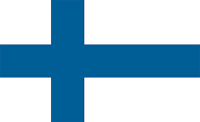







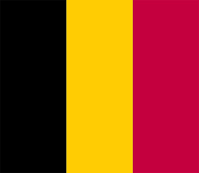

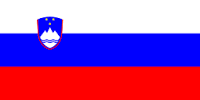





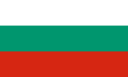


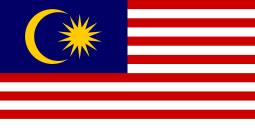



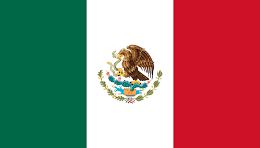
