Let's consider having a dataset X ∈ ℜM × N (M N-dimensional samples) drawn from a multivariate data generating process pdata. The goal of the mean shift algorithm applied to a clustering problem is to find the regions where pdata is maximum and associate the samples contained in a surrounding subregion to the same cluster. As pdata is a Probability Density Function (PDF), it is reasonable for representing it as the sum of regular PDFs (for example, Gaussians) characterized by a small subset of parameters, such as mean and variance. In this way, a sample can be supposed to be generated by the PDF with the highest probability. We are going to discuss this process also in Chapter 5, Soft Clustering and Gaussian Mixture Models, and Chapter 6, Anomaly Detection. For our purposes, it's helpful to restructure the problem as an iterative procedure...




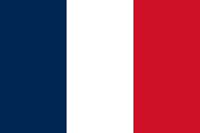


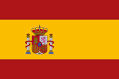



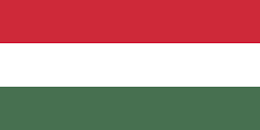

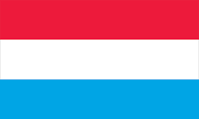





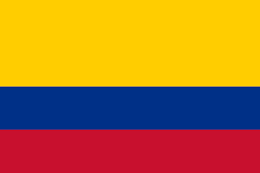
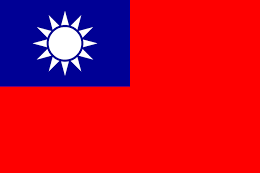
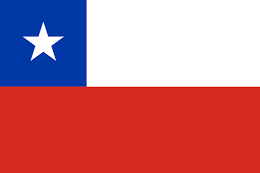



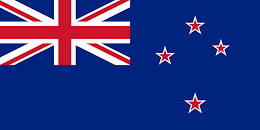
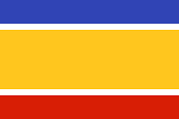
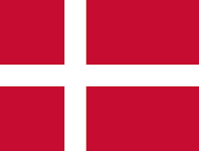
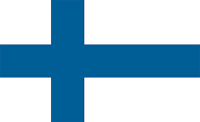







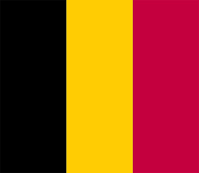

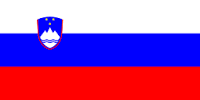





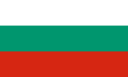


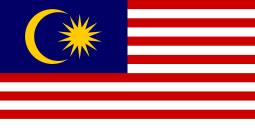



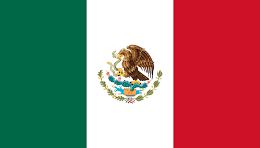
