



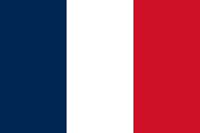


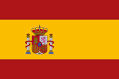



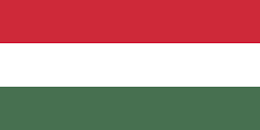

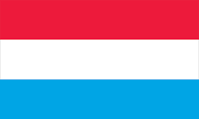





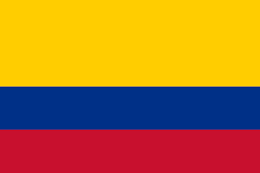
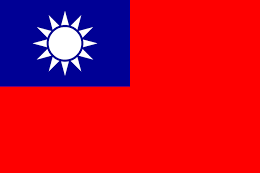
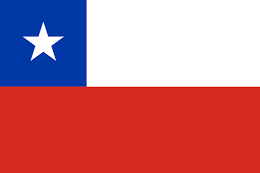



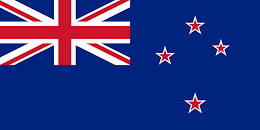
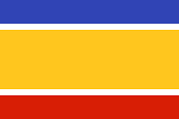
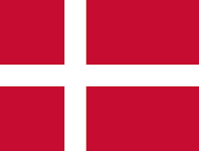
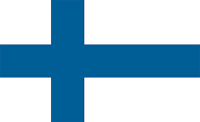







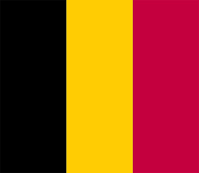

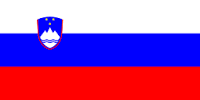





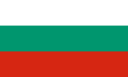


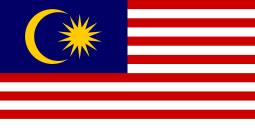



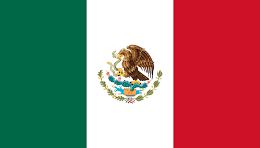

The rise of big data and business intelligence has been one of the hottest topics to hit the tech world. Everybody who’s anybody has heard of the term business intelligence, yet very few can actually articulate what this means. Nonetheless it’s something all organizations are demanding. But you must be wondering why and how do you develop business intelligence? Enter data scientists!
The concept of data science was developed to work with large sets of structured and unstructured data. So what does this mean?
Let me explain. Data science was introduced to explore and give meaning to random sets of data floating around (we are talking about huge quantities here, that is, terabytes and petabytes), which are then used to analyze and help identify areas of poor performance, areas of improvement, and areas to capitalize on.
The concept was introduced for large data-driven organisations that required consultants and specialists to deal with complex sets of data. However, data science has been adopted very quickly by organizations of all shapes and sizes, so naturally an element of flexibility would be required to fit data scientists in the modern work flow.
There seems to be a shortage for data scientists and an increase in the amount of data out there. The modern data scientist is one who would be able to apply analytical skills necessary to any organization with or without large sets of data available. They are required to carry out data mining tasks to discover relevant meaningful data.
Yet, smaller organizations wouldn’t have enough capital to invest in paying for a person who is experienced enough to derive such results. Nonetheless, because of the need for information, they might instead turn to a general data analyst and help them move towards data science and provide them with tools/processes/frameworks that allow for the rapid prototyping of models instead.
The natural flow of work would suggest data analysis comes after data mining, and in my opinion analysis is at the heart of the data science. Learning languages like R and Python are fundamental to a good data scientist’s tool kit. However would a data scientist with a background in mathematics and statistics and little to no knowledge of R and Python still be as efficient?
Now, the way I see it, data science is composed of four key topic areas crucial to achieving business intelligence, which are data mining, data analysis, data visualization, and machine learning.
Data analysis can be carried out in many forms; it’s essentially looking at data and understanding it to make a factual conclusion from it (in simple terms). A data scientist may choose to use Microsoft Excel and VBA to analyze their data, but it wouldn’t be as accurate, clean, or as in depth as using Python or R, but it sure would be useful as a quick win with smaller sets of data.
The approach here is that starting with something like Excel doesn’t mean it’s not counted as data science, it’s just a different form of it, and more importantly it actually gives a good foundation to progress on to using things like MySQL, R, Julia, and Python, as with time, business needs would grow and so would expectations of the level of analysis. In my opinion, a good data scientist is not one who knows more than one or two languages or tools, but one who is well-versed in the majority of them and knows which language and skill set are best suited to the task in hand.
Data visualization is hugely important, as numbers themselves tell a story, but when it comes to representing the data to customers or investors, they're going to want to view all the different aspects of that data as quickly and easily as possible. Graphically representing complex data is one of the most desirable methods, but the way the data is represented varies dependent on the tool used, for example R’s GGplot2 or Python’s Matplotlib. Whether you’re working for a small organization or a huge data-driven company, data visualization is crucial.
The world of artificial intelligence introduced the concept of machine learning, which has exploded on the scene and to an extent is now fundamental to large organizations. The opportunity for organizations to move forward by understanding a consumer’s behaviour and equally matching their expectations has never been so valuable. Data scientists are required to learn complex algorithms and core concepts such as classifications, recommenders, neural networks, and supervised and unsupervised learning techniques. This is just touching the edges of this exciting field, which goes into much more depth especially with emerging concepts such as deep learning.
To conclude, we covered the basic fundamentals of data science and what it means to be data scientists. For all you R and Python developers (not forgetting any mathematical wizards out there), data science has been described as the ‘Sexiest job of 21st century’ as well as being handsomely rewarding too. The rise in jobs for data scientists has without question exploded and will continue to do so; according to global management firm McKinsey & Company, there will be a shortage of 140,000 to 190,000 data scientists due to the continued rise of ‘big data’.