Placeholders and variables are key tools in with regard to using computational graphs in TensorFlow. We must understand the difference between them and when to best use them to our advantage.
Using placeholders and variables
Getting ready
One of the most important distinctions to make with data is whether it is a placeholder or a variable. Variables are the model parameters of the algorithm, and TensorFlow keeps track of how to change these to optimize the algorithm. Placeholders are objects that allow you to feed in data of a specific type and shape, or that depend on the results of the computational graph, such as the expected outcome of a computation.
How to do it...
The main way to create a variable is by using the Variable() function, which takes a tensor as an input and outputs a variable. This is only the declaration, and we still need to initialize the variable. Initializing is what puts the variable with the corresponding methods on the computational graph. Here is an example of creating and initializing a variable:
my_var = tf.Variable(tf.zeros([2,3])) sess = tf.Session() initialize_op = tf.global_variables_initializer() sess.run(initialize_op)
To see what the computational graph looks like after creating and initializing a variable, see the following part of this recipe. Placeholders are just holding the position for data to be fed into the graph. Placeholders get data from a feed_dict argument in the session. To put a placeholder into the graph, we must perform at least one operation on the placeholder. In the following code snippet, we initialize the graph, declare x to be a placeholder (of a predefined size), and define y as the identity operation on x, which just returns x. We then create data to feed into the x placeholder and run the identity operation. The code is shown here, and the resultant graph is in the following section:
sess = tf.Session() x = tf.placeholder(tf.float32, shape=[2,2]) y = tf.identity(x) x_vals = np.random.rand(2,2) sess.run(y, feed_dict={x: x_vals}) # Note that sess.run(x, feed_dict={x: x_vals}) will result in a self-referencing error.
How it works...
The computational graph of initializing a variable as a tensor of zeros is shown in the following diagram:
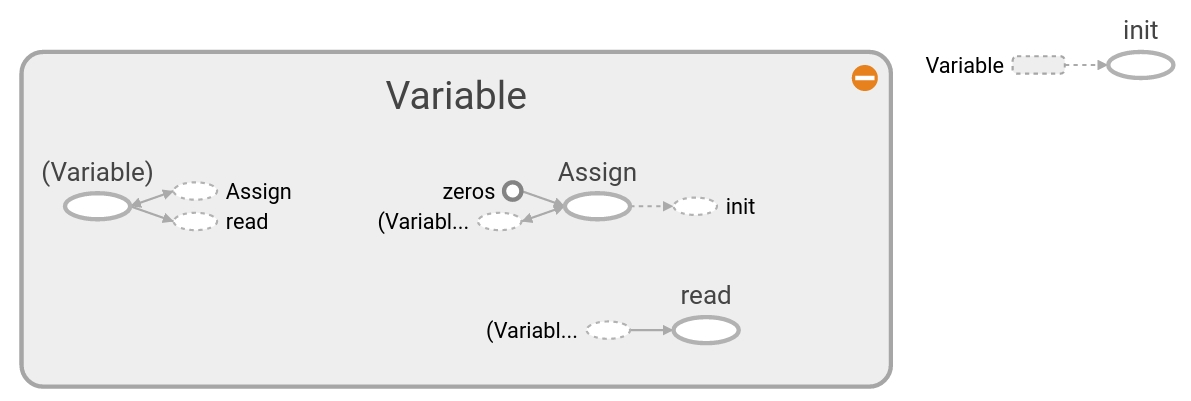
Here, we can see what the computational graph looks like in detail with just one variable, initialized all to zeros. The grey shaded region is a very detailed view of the operations and constants that were involved. The main computational graph with less detail is the smaller graph outside of the grey region in the upper-right corner. For more details on creating and visualizing graphs, see the first section of Chapter 10, Taking TensorFlow to Production. Similarly, the computational graph of feeding a NumPy array into a placeholder can be seen in the following diagram:
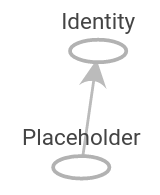
The grey shaded region is a very detailed view of the operations and constants that were involved. The main computational graph with less detail is the smaller graph outside of the grey region in the upper-right corner.
There's more...
During the run of the computational graph, we have to tell TensorFlow when to initialize the variables we have created. While each variable has an initializer method, the most common way to do this is with the helper function, that is, global_variables_initializer(). This function creates an operation in the graph that initializes all the variables we have created, as follows:
initializer_op = tf.global_variables_initializer()
But if we want to initialize a variable based on the results of initializing another variable, we have to initialize variables in the order we want, as follows:
sess = tf.Session() first_var = tf.Variable(tf.zeros([2,3])) sess.run(first_var.initializer) second_var = tf.Variable(tf.zeros_like(first_var)) # 'second_var' depends on the 'first_var' sess.run(second_var.initializer)