When dealing with complex topics, we usually end up with a dataset with a large number of variables. To find meaning in this kind of dataset is typically a tricky task. Luckily, there are some analytical techniques that can help us. One of those techniques is principal component analysis (PCA), which is a data reduction technique. Mathematical transformation in this analysis enables us to derive the most informative dimensions of our dataset. The mathematics underlying the analysis singular value decomposition (SVD) is somewhat complex, so we won't go into too much detail in this recipe. The basics of PCA can be described like this: you start with a dataset with many variables, then you simplify that dataset by turning your original variables into a smaller number of principal components in a way that guarantees that you&apos...




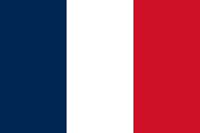


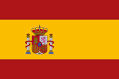



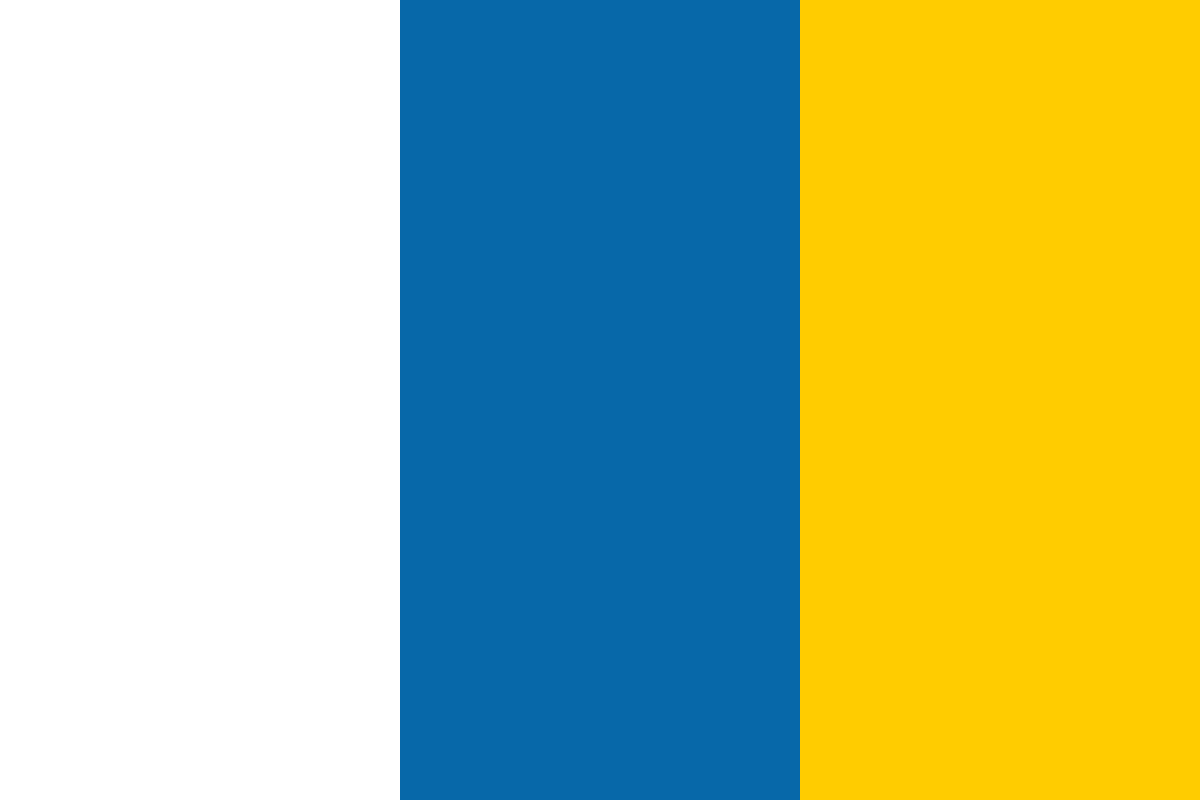
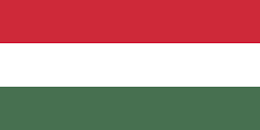

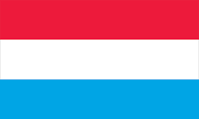





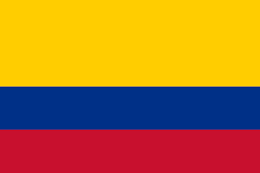
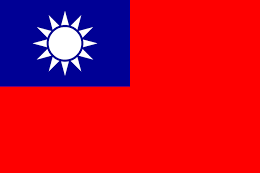
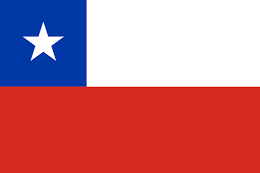



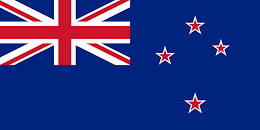
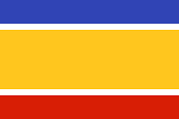
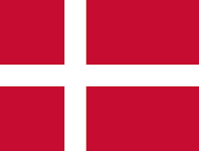
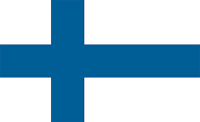







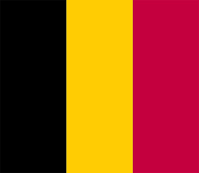

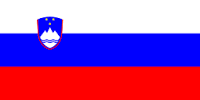





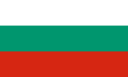


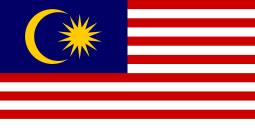



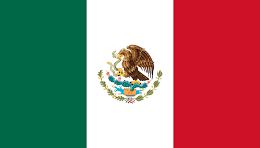
