We are already aware that RNNs are cyclical graphs, unlike feedforward networks, which are acyclic directional graphs. In feedforward networks, the error derivatives are calculated from the layer above. However, in an RNN we don't have such layering to perform error derivative calculations. A simple solution to this problem is to unroll the RNN and make it similar to a feedforward network. To enable this, the hidden units from the RNN are replicated at each time step. Each time step replication forms a layer that is similar to layers in a feedforward network. Each time step t layer connects to all possible layers in the time step t+1. Therefore, we randomly initialize the weights, unroll the network, and then use backpropagation to optimize the weights in the hidden layer. The lowest layer is initialized by passing parameters. These parameters...




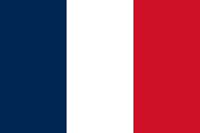


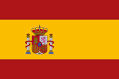




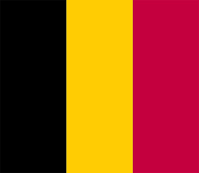
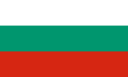
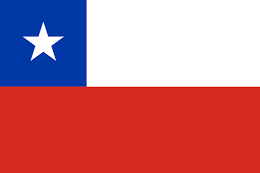
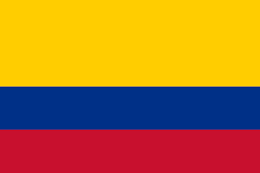
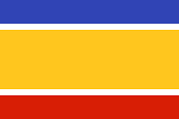

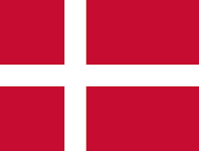



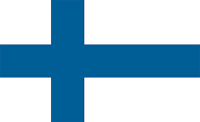

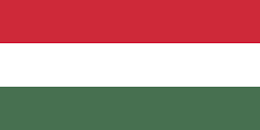






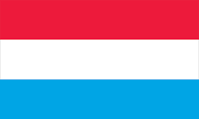
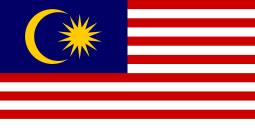

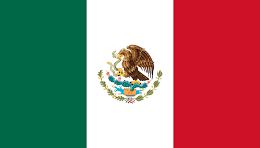

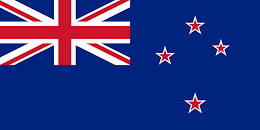







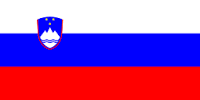




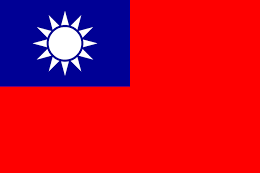


