In this section, we'll talk about arguably the most popular generative model today: the GANs framework. It was first introduced in 2014 in the landmark paper Generative Adversarial Nets(http://papers.nips.cc/paper/5423-generative-adversarial-nets.pdf) by Ian J. Goodfellow, Jean Pouget-Abadie, Mehdi Mirza, Bing Xu, David Warde-Farley, Sherjil Ozair Aaron Courville, and Yoshua Bengio. The GANs framework can work with any type of data, but it's most popular application by far is to generate images, and we'll discuss them in this context only. Let's see how it work:
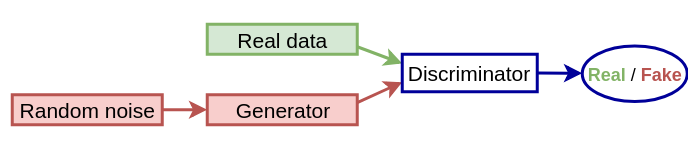
A GAN system
A GAN is a system of two components (neural networks):
- Generator: This is the generative model itself. It takes a probability distribution (random noise) as input and tries to generate a realistic output image. Its purpose is similar to the decoder part...