Sanger's network
A Sanger's network is a neural network model for online principal component extraction, proposed by T. D. Sanger in Optimal Unsupervised Learning in Sanger T. D., Single-Layer Linear Feedforward Neural Network, Neural Networks, 1989/2. The author started with the standard version of Hebb's rule and modified it to be able to extract a variable number of principal components in descending order
. The resulting approach, which is a natural extension of Oja's rule, has been called the Generalized Hebbian Rule (GHA)—you might also sometimes see it called Generalized Hebbian Learning (GHL). The structure of the network is represented in the following diagram:
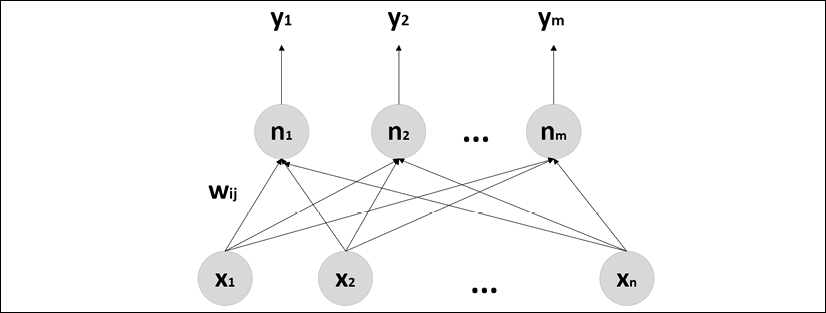
Structure of a Sanger's Network
The network is fed with samples extracted from an n-dimensional dataset:

The m output neurons are connected to the input through a weight matrix, W = {wij}, where the first index refers to the input components (pre-synaptic units) and...