In many cases, the dimensionality of the input dataset X is high and so is the complexity of every related machine learning algorithm. Moreover, the information is seldom spread uniformly across all the features and, as discussed in the previous chapter, Chapter 2, Important Elements in Machine Learning, there will be high-entropy features together with low-entropy ones, which, of course, don't contribute dramatically to the final outcome. This concept can also be expressed by considering a fundamental assumption of semi-supervised learning, called the manifold assumption. It states (without a formal proof, as it's an empirical hypothesis) that data with high dimensionality normally lies on lower-dimensional manifolds. If the reader is not familiar with the concept of a manifold, it's not necessary for our purpose to provide a complete...




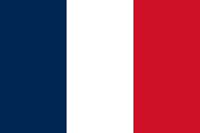


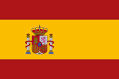



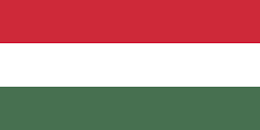

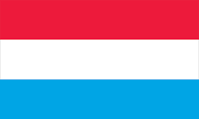





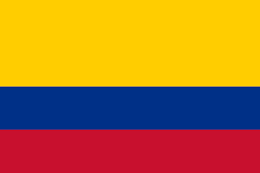
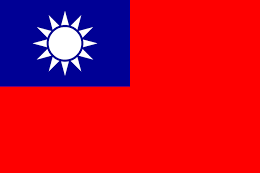
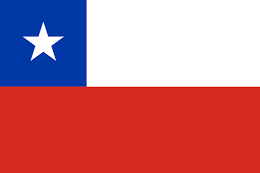



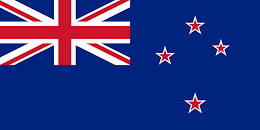
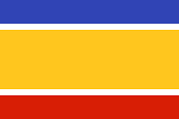
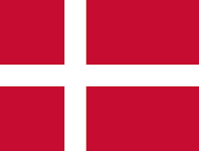
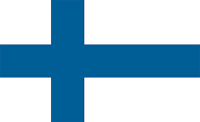







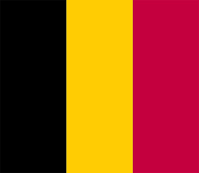

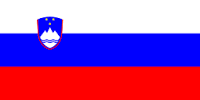





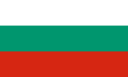


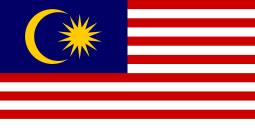



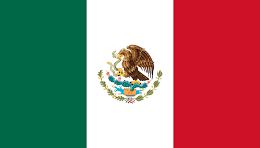
