In this chapter, we took a very close look at how the agents in ML-Agents perceive their environment and process input. An agent's perception of the environment is completely in control by the developer, and it is often a fine balance of how much or how little input/state you want to give an agent. We played with many examples in this chapter and started by taking an in-depth look at the Hallway sample and how an agent uses rays to perceive objects in the environment. Then, we looked at how an agent can use visual observations, not unlike us humans, as input or state that it may learn from. From this, we delved into the CNN architecture that ML-Agents uses to encode the visual observations it provides to the agent. We then learned how to modify this architecture by adding or removing convolution or pooling layers. Finally, we looked at the role of memory, or how recurrent...




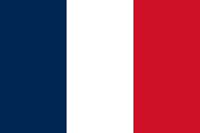


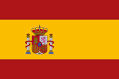




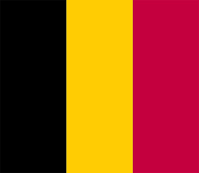
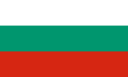
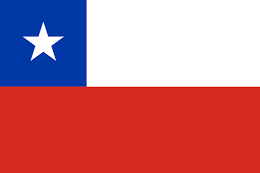
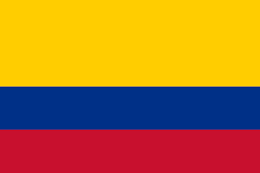
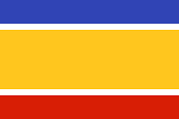

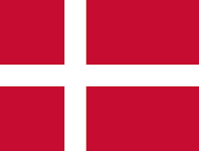



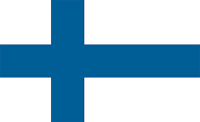

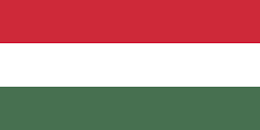






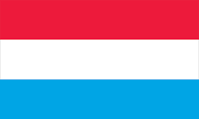
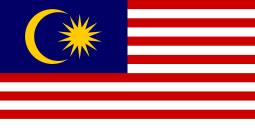

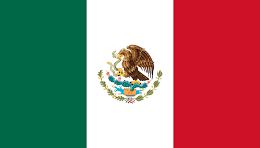

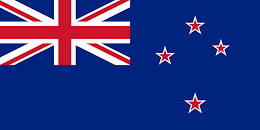







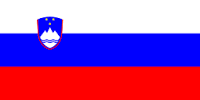




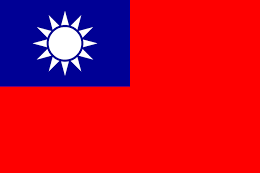


