Chapter 9: A practical NLP project workflow in an organisation
Code for LSTM model
- Check if GPU is detected
import tensorflow as tf
tf.test.gpu_device_name()
- Setting up collar notebook
from google.colab import drive
drive.mount('/content/gdrive')
# Run the below command in a new cell
cd /content/gdrive/My Drive/Lesson-9/
# Run the below command in a new cell
!unzip data.csv.zip
- Import necessary Python packages and classes.
import os
import re
import pickle
import pandas as pd
from keras.preprocessing.text import Tokenizer
from keras.preprocessing.sequence import pad_sequences
from keras.models import Sequential
from keras.layers import Dense, Embedding, LSTM
- Load the data file.
def preprocess_data(data_file_path):
data = pd.read_csv(data_file_path, header=None) # read the csv
data.columns = ['rating', 'title', 'review'] # add column names
data['review'] = data['review'].apply(lambda x: x.lower()) # change all text to lower
data['review'] = data['review'].apply((lambda x: re.sub('[^a-zA-z0-9\s]','',x))) # remove all numbers
return data
df = preprocess_data('data.csv')
- Initialize tokenization.
max_features = 2000
maxlength = 250
tokenizer = Tokenizer(num_words=max_features, split=' ')
- Fit tokenizer.
tokenizer.fit_on_texts(df['review'].values)
X = tokenizer.texts_to_sequences(df['review'].values)
- Pad sequences.
X = pad_sequences(X, maxlen=maxlength)
- Get target variable
y_train = pd.get_dummies(df.rating).values
embed_dim = 128
hidden_units = 100
n_classes = 5
model = Sequential()
model.add(Embedding(max_features, embed_dim, input_length = X.shape[1]))
model.add(LSTM(hidden_units))
model.add(Dense(n_classes, activation='softmax'))
model.compile(loss = 'categorical_crossentropy', optimizer='adam',metrics = ['accuracy'])
print(model.summary())
- Fit the model.
model.fit(X[:100000, :], y_train[:100000, :], batch_size = 128, epochs=15, validation_split=0.2)
- Save model and tokenizer.
model.save('trained_model.h5') # creates a HDF5 file 'trained_model.h5'
with open('trained_tokenizer.pkl', 'wb') as f: # creates a pickle file 'trained_tokenizer.pkl'
pickle.dump(tokenizer, f)
from google.colab import files
files.download('trained_model.h5')
files.download('trained_tokenizer.pkl')
Code for Flask
- Import the necessary Python packages and classes.
import re
import pickle
import numpy as np
from flask import Flask, request, jsonify
from keras.models import load_model
from keras.preprocessing.sequence import pad_sequences
- Define the input files and load in dataframe
def load_variables():
global model, tokenizer
model = load_model('trained_model.h5')
model._make_predict_function() # https://github.com/keras-team/keras/issues/6462
with open('trained_tokenizer.pkl', 'rb') as f:
tokenizer = pickle.load(f)
- Define preprocessing functions similar to the training code:
def do_preprocessing(reviews):
processed_reviews = []
for review in reviews:
review = review.lower()
processed_reviews.append(re.sub('[^a-zA-z0-9\s]', '', review))
processed_reviews = tokenizer.texts_to_sequences(np.array(processed_reviews))
processed_reviews = pad_sequences(processed_reviews, maxlen=250)
return processed_reviews
- Define a Flask app instance:
app = Flask(__name__)
- Define an endpoint that displays a fixed message:
@app.route('/')
def home_routine():
return 'Hello World!'
- We'll have a prediction endpoint, to which we can send our review strings. The kind of HTTP request we will use is a 'POST' request:
@app.route('/prediction', methods=['POST'])
def get_prediction():
# get incoming text
# run the model
if request.method == 'POST':
data = request.get_json()
data = do_preprocessing(data)
predicted_sentiment_prob = model.predict(data)
predicted_sentiment = np.argmax(predicted_sentiment_prob, axis=-1)
return str(predicted_sentiment)
- Start the web server.
if __name__ == '__main__':
# load model
load_variables()
app.run(debug=True)
- Save this file as app.py (any name could be used). Run this code from the terminal using app.py:
python app.py
The output is as follows:
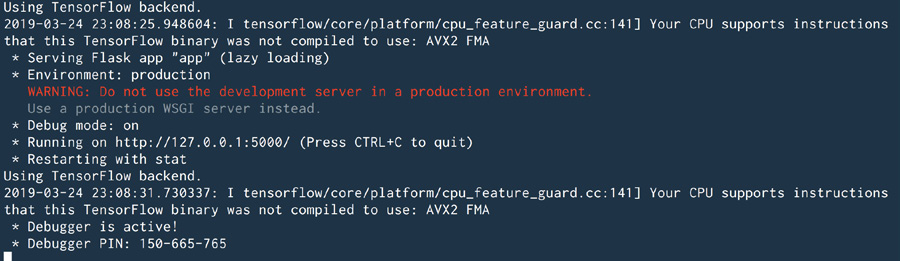