We will begin our implementation with a simple MLP-based model, that is, our generator and discriminator will be dense, fully connected, networks. Then, we will move on to implementing a convolutional GAN.
An MLP model
We will now focus in creating the model shown in Figure 14.3. The model has a generator and discriminator that are distinct in terms of their numbers of layers and total parameters. It is usually the case that the generator takes more resources to build than the discriminator. This is intuitive if you think about it: the creative process is usually more complex than the process of recognition. In life, it might be easy to recognize a painting from Pablo Picasso if you see all of his paintings repeatedly.
However, it might be much harder, in comparison, to actually paint like Picasso:
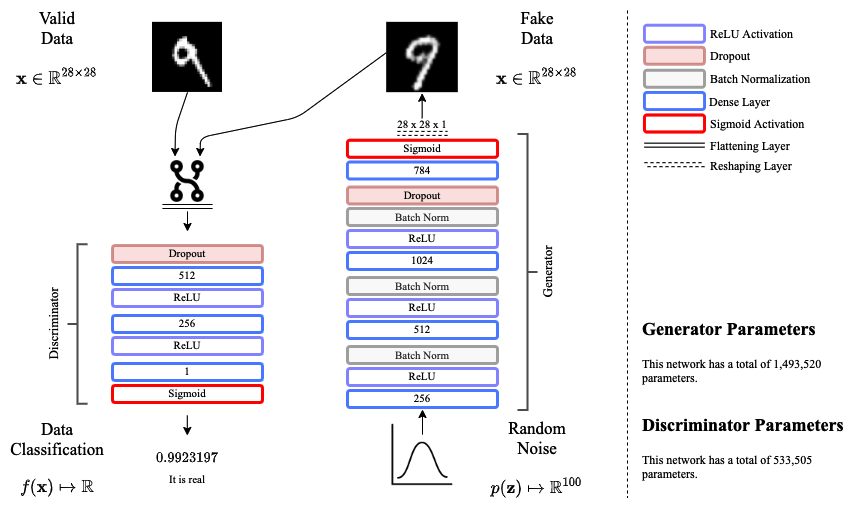
This figure depicts an icon that simply represents the fact that the discriminator will be taking both fake and valid data and learning...