Using logistic regression
Contrary to its name, logistic regression is a classification method, and is very powerful when it comes to text-based classification. It achieves this by first performing regression on a logistic function, hence the name.
A bit of math with a small example
To get an initial understanding of the way logistic regression works, let us first take a look at the following example, where we have an artificial feature value at the X axis plotted with the corresponding class range, either 0 or 1. As we can see, the data is so noisy that classes overlap in the feature value range between 1 and 6. Therefore, it is better to not directly model the discrete classes, but rather the probability that a feature value belongs to class 1, P(X). Once we possess such a model, we could then predict class 1 if P(X) > 0.5 or class 0 otherwise:
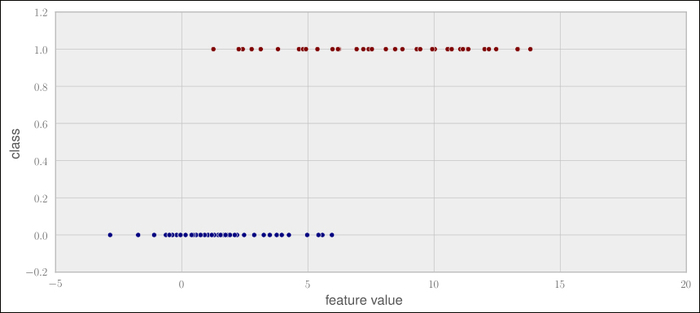
Mathematically, it is always difficult to model something that has a finite range, as is the case here with our discrete labels 0 and 1. We can...