



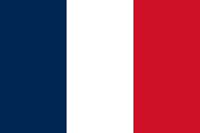


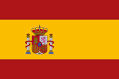




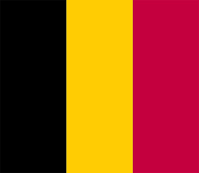
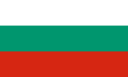
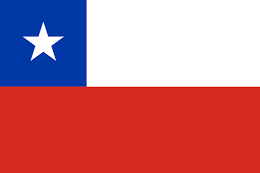
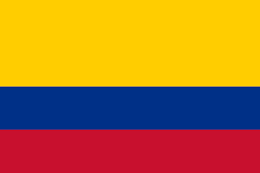
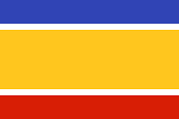

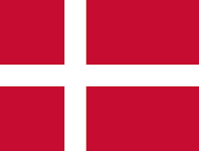



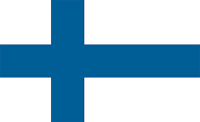

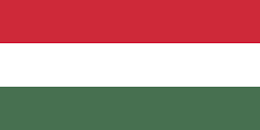






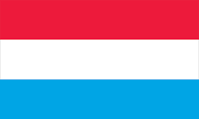
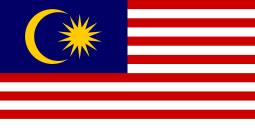

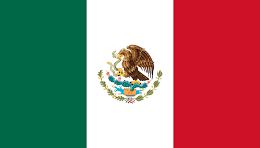

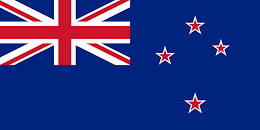







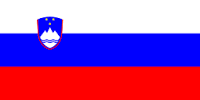




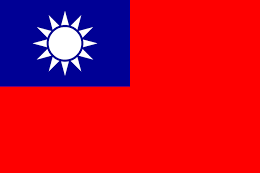



Recently, researchers from DeepMind released their research where they designed AI agents that can team up to play Quake III Arena’s Capture the Flag mode. The highlight of this research is, these agents were able to team up against human players or play alongside them, tailoring their behavior accordingly.
We have previously seen instances of an AI agent beating humans in video games like StarCraft II and Dota 2. However, these games did not involve agents playing in a complex environment or required teamwork and interaction between multiple players.
In their research paper titled, “Human-level performance in 3D multiplayer games with population-based reinforcement learning”, a group of 30 AIs were collectively trained to play five-minute rounds of Capture the Flag, a game mode in which teams must retrieve flags from their opponents while retaining their own.
https://youtu.be/OjVxXyp7Bxw
While playing the rounds in Capture the Flag the DeepMind AI was able to outperform human teammates, with the reaction time slowed down to that of a typical human player. Rather than a number of AIs teaming up on a group of human players in a game of Dota 2, the AI was able to play alongside them as well.
Using Reinforcement learning, the AI taught itself the skill which helped it to pick up the rules of the game over thousands of matches in randomly generated environments.
“No one has told [the AI] how to play the game — only if they’ve beaten their opponent or not. The beauty of using [an] approach like this is that you never know what kind of behaviors will emerge as the agents learn,” said Max Jaderberg, a research scientist at DeepMind who recently worked on AlphaStar, a machine learning system that recently bested a human team of professionals at StarCraft II.
Greg Brockman, a researcher at OpenAI told The New York Times, “Games have always been a benchmark for A.I. If you can’t solve games, you can’t expect to solve anything else.”
According to The New York Times, “such skills could benefit warehouse robots as they work in groups to move goods from place to place, or help self-driving cars navigate en masse through heavy traffic.”
Talking about limitations, the researchers say, “Limitations of the current framework, which should be addressed in future work, include the difficulty of maintaining diversity in agent populations, the greedy nature of the meta-optimization performed by PBT, and the variance from temporal credit assignment in the proposed RL updates.”
“Our work combines techniques to train agents that can achieve human-level performance at previously insurmountable tasks. When trained in a sufficiently rich multiagent world, complex and surprising high-level intelligent artificial behavior emerged”, the paper states.
To know more about this news in detail, visit the official research paper on Science.
OpenAI Five beats pro Dota 2 players; wins 2-1 against the gamers
Samsung AI lab researchers present a system that can animate heads with one-shot learning
Amazon is reportedly building a video game streaming service, says Information