




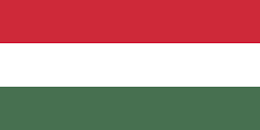

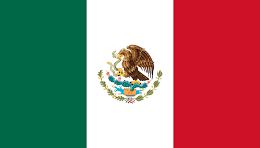


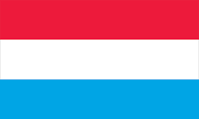



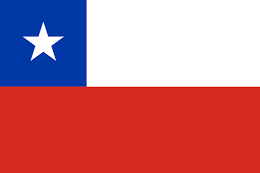



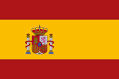


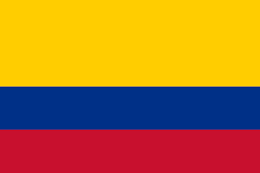
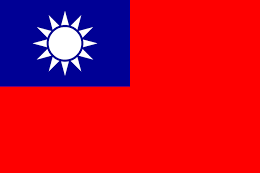


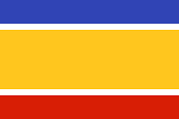
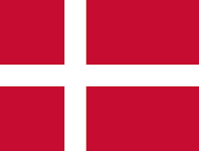
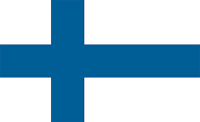



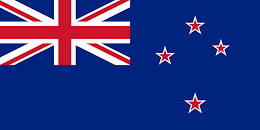


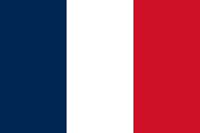



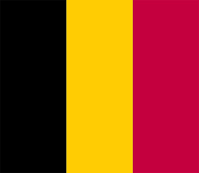

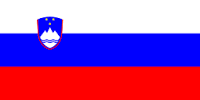




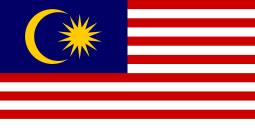


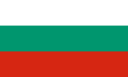




This article is an excerpt from the book Creators of Intelligence, by Dr. Alex Antic. This book will provide you with insights from 18 AI leaders on how to build a rewarding data science career.
As Generative Artificial Intelligence (AI) continues to advance, the need for ethical considerations becomes increasingly vital. In this article, we engage in a conversation between a Generative AI expert, Edward Santow, and an author to uncover practical ways to incorporate ethics into the rapidly evolving landscape of generative AI, ensuring its responsible and beneficial implementation.
Generative AI is a rapidly developing field with the potential to revolutionize many aspects of our lives. However, it also raises a number of ethical concerns. Some of the most pressing ethical issues in generative AI include:
It is important to address these ethical concerns in order to ensure that generative AI is used in a responsible and ethical manner. Some of the steps that can be taken to address these concerns include:
By addressing these ethical concerns, we can help to ensure that generative AI is used in a responsible and ethical manner.
Ed Santow: In one sense, it hasn’t, as the frameworks are broad enough and apply to AI generally, and their application depends on adapting to the specific context in which they’re being applied.
One of the great advantages of this is that generative AI is included within its scope. It may be a newer form of AI, as compared with analytical AI, but existing AI ethics frameworks already cover a range of privacy and human rights issue, so they are applicable. The previous work to create those frameworks has made it easier and faster to adapt to the specific aspects of generative AI from an ethical perspective.
One of the main complexities is the relatively low community understanding of how generative AI actually works and, particularly, the science behind it. Very few people can distinguish between analytical and generative AI. Most people in senior roles haven’t made the distinction yet or identified the true impact.
The issue is, if you don’t understand the underlying technology well enough, then it’s difficult to make the frameworks work in practice.
Analytical and generative AI share similar core science. However, generative AI can pose greater risks than simple classification AI. But the nature and scale of those risks generally haven’t been worked through in most organizations. Simply setting black-and-white rules – such as you can or can’t use generative AI – isn’t usually the best answer. You need to understand how to safely use it.
Ed Santow: First and foremost, they need to understand that skills and knowledge are vital. They need to upskill their staff and develop a better understanding of the technology and its implications – and this applies at all levels of the organization.
Second, they need to set a nuanced policy framework, outline how to use such technology safely and develop appropriate risk mitigation procedures that can flag when it’s not safe to rely on the outputs of generative AI applications. Most AI ethics frameworks don’t go into this level of detail.
Finally, consideration needs to be given to how generative AI can be used lawfully. For example, entering confidential client data – or proprietary company data – into ChatGPT is likely to be unlawful, yet we also know this is happening.
Edward Santow: There are simply no shortcuts. People can’t assume that even though others in their industry are using generative AI, their organization can use it without considering the legal and ethical ramifications.
They also need to be able to experiment safely with such technology. For example, a new chatbot based on generative AI shouldn’t be simply unleased on customers. They need to first test and validate it in a controlled environment to understand all the risks – including the ethical and legal ramifications.
Leaders need to ensure that an appropriately safe test environment is established to mitigate any risk of harm to staff or customers.
In this article, we went through various ethical issues that can arise while implementing Generative AI and some ways to tackle these challenges effectively. We also learned certain practical best practices through an expert opinion from an expert in the field of Generative AI.
Dr. Alex Antic is an award-winning Data Science and Analytics Leader, Consultant, and Advisor, and a highly sought Speaker and Trainer, with over 20 years of experience. Alex is the CDO and co-founder of Healices Health - which focuses on advancing cancer care using Data Science and is co-founder of Two Twigs - a Data Science consulting, advisory, and training company. Alex has been described as "one of Australia’s iconic data leaders" and "one of the most premium thought leaders in data analytics globally". He was recognized in 2021 as one of the Top 5 Analytics Leaders by the Institute of Analytics Professionals of Australia (IAPA). Alex is an Adjunct Professor at RMIT University, and his qualifications include a Ph.D. in Applied Mathematics.