



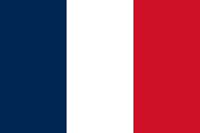


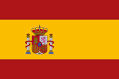



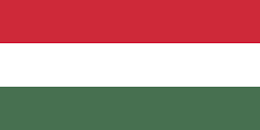

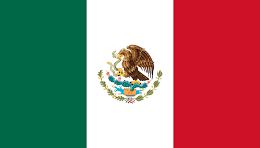


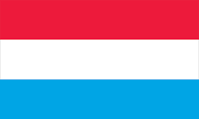



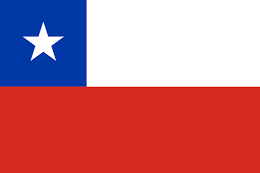


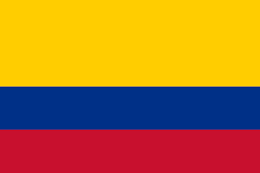
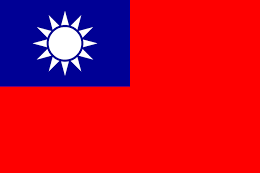


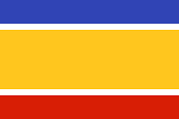
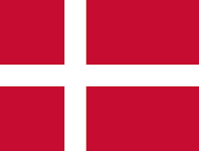
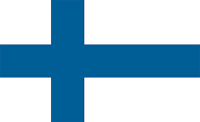



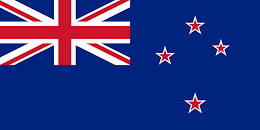





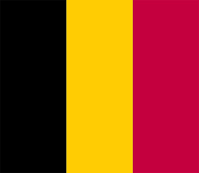

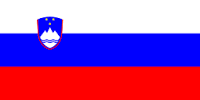




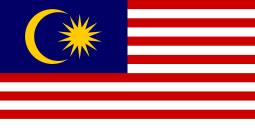


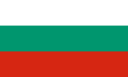



In order to begin the discussion on computer vision, observe the following image:
Even if we have never done this activity before, we can clearly tell that the image is of
people skiing in the snowy mountains on a cloudy day. This information that we perceive is
quite complex and can be subdivided into more basic inferences for a computer vision
System.
The most basic observation that we can get from an image is of the things or objects in it. In the previous image, the various things that we can see are trees, mountains, snow, sky,
people, and so on. Extracting this information is often referred to as image classification,
where we would like to label an image with a predefined set of categories. In this case, the
labels are the things that we see in the image.
A wider observation that we can get from the previous image is landscape. We can tell that
the image consists of snow, mountains, and sky, as shown in the following image:
Although it is difficult to create exact boundaries for where the snow, mountain, and sky are in the image, we can still identify approximate regions of the image for each of them. This is often termed as segmentation of an image, where we break it up into regions according to object occupancy.
Making our observation more concrete, we can further identify the exact boundaries of objects in the image, as shown in the following figure:
In the image, we see that people are doing different activities and as such have different
shapes; some are sitting, some are standing, some are skiing. Even with this many
variations, we can detect objects and can create bounding boxes around them. Only a few
bounding boxes are shown in the image for understanding—we can observe much more
than these.
While, in the image, we show rectangular bounding boxes around some objects, we are not
categorizing what object is in the box. The next step would be to say the box contains a
person. This combined observation of detecting and categorizing the box is often referred to as object detection.
Extending our observation of people and surroundings, we can say that different people in the image have different heights, even though some are nearer and others are farther from the camera. This is due to our intuitive understanding of image formation and the relations of objects. We know that a tree is usually much taller than a person, even if the trees in the image are shorter than the people nearer to the camera. Extracting the information about geometry in the image is another sub-field of computer vision, often referred to as image reconstruction.
In the previous section, we developed an initial understanding of computer vision. With
this understanding, there are several algorithms that have been developed and are used in
industrial applications. Studying these not only improve our understanding of the system
but can also seed newer ideas to improve overall systems.
In this section, we will extend our understanding of computer vision by looking at various
applications and their problem formulations:
The field is quickly evolving, not only through making newer methods of image analysis
but also finding newer applications where computer vision can be used. Therefore,
applications are not just limited to those explained above.
[box type="note" align="" class="" width=""]Check out this post on Image filtering techniques in OpenCV.[/box]
In this section, we will see basic image operations for reading and writing images. We will
also, see how images are represented digitally. Before we proceed further with image IO, let's see what an image is made up of in the digital world.
An image is simply a two-dimensional array, with each cell of the array containing intensity values. A simple image is a black and white image with 0s representing white and 1s representing black. This is also referred to as a binary image. A further extension of this is dividing black and white into a broader grayscale with a range of 0 to An image of this type, in the three-dimensional view, is as follows, where x and y are pixel locations and z is the intensity value:
This is a top view, but on viewing sideways we can see the variation in the intensities that make up the image:
We can see that there are several peaks and image intensities that are not smooth. Let's apply smoothing algorithm.
As we can see, pixel intensities form more continuous formations, even though there is no
significant change in the object representation.
import numpy as np
import matplotlib.pyplot as plt
from mpl_toolkits.mplot3d import Axes3D
import cv2
# loads and read an image from path to file
img = cv2.imread('../figures/building_sm.png')
# convert the color to grayscale
gray = cv2.cvtColor(img, cv2.COLOR_BGR2GRAY)
# resize the image(optional)
gray = cv2.resize(gray, (160, 120))
# apply smoothing operation
gray = cv2.blur(gray,(3,3))
# create grid to plot using numpy
xx, yy = np.mgrid[0:gray.shape[0], 0:gray.shape[1]]
# create the figure
fig = plt.figure()
ax = fig.gca(projection='3d')
ax.plot_surface(xx, yy, gray ,rstride=1, cstride=1, cmap=plt.cm.gray,
linewidth=1)
# show it
plt.show()
This code uses the following libraries: NumPy, OpenCV, and matplotlib. In the further sections of this article, we will see operations on images using their color properties. Please download the relevant images from the website to view them clearly.
We can use the OpenCV library to read an image, as follows. Here, change the path to the
image file according to use:
import cv2
# loads and read an image from path to file
img = cv2.imread('../figures/flower.png')
# displays previous image
cv2.imshow("Image",img)
# keeps the window open until a key is pressed
cv2.waitKey(0)
# clears all window buffers
cv2.destroyAllWindows()
The resulting image is shown in the following screenshot:
Here, we read the image in BGR color format where B is blue, G is green, and R is red. Each pixel in the output is collectively represented using the values of each of the colors. An example of the pixel location and its color values is shown in the previous figure bottom.
An image is made up pixels and is usually visualized according to the value stored. There is also an additional property that makes different kinds of image. Each of the value stored in a pixel is linked to a fixed representation. For example, a pixel value of 10 can represent gray intensity value 1o or blue color intensity value 10 and so on. It is therefore important to understand different color types and their conversion. In this section, we will see color types and conversions using OpenCV.
[box type="note" align="" class="" width=""]Did you know OpenCV 4 is on schedule for July release, check out this news piece to know about it in detail.[/box]
Grayscale: This is a simple one channel image with values ranging from 0 to 255 that represent the intensity of pixels. The previous image can be converted to grayscale, as follows:
import cv2
# loads and read an image from path to file
img = cv2.imread('../figures/flower.png')
# convert the color to grayscale
gray = cv2.cvtColor(img, cv2.COLOR_BGR2GRAY)
# displays previous image
cv2.imshow("Image",gray)
# keeps the window open until a key is pressed
cv2.waitKey(0)
# clears all window buffers
cv2.destroyAllWindows()
The resulting image is as shown in the following screenshot:
HSV and HLS: These are another representation of color representing H is hue, S is saturation, V is value, and L is lightness. These are motivated by the human perception system. An example of image conversion for these is as follows:
# convert the color to hsv
hsv = cv2.cvtColor(img, cv2.COLOR_BGR2HSV)
# convert the color to hls
hls = cv2.cvtColor(img, cv2.COLOR_BGR2HLS)
This conversion is as shown in the following figure, where an input image read in BGR
format is converted to each of the HLS (on left) and HSV (on right) colortypes:
LAB color space: Denoted L for lightness, A for green-red colors, and B for blueyellow colors, this consists of all perceivable colors. This is used to convert between one type of colorspace (for example, RGB) to others (such as CMYK) because of its device independence properties. On devices where the format is different to that of the image that is sent, the incoming image color space is first converted to LAB and then to the corresponding space available on the device. The output of converting an RGB image is as follows:
This article is an excerpt from the book Practical Computer Vision written by Abhinav Dadhich. This book will teach you different computer vision techniques and show how to apply them in practical applications.