Consider that you are teaching the dog to catch a ball, but you cannot teach the dog explicitly to catch a ball; instead, you will just throw a ball, and every time the dog catches the ball, you will give it a cookie. If it fails to catch the ball, you will not give a cookie. The dog will figure out what actions made it receive a cookie and will repeat those actions.
Similarly, in a RL environment, you will not teach the agent what to do or how to do instead, you will give a reward to the agent for each action it does. The reward may be positive or negative. Then the agent will start performing actions which made it receive a positive reward. Thus, it is a trial and error process. In the previous analogy, the dog represents the agent. Giving a cookie to the dog upon catching the ball is a positive reward, and not giving a cookie is a negative reward.
There might be delayed rewards. You may not get a reward at each step. A reward may be given only after the completion of a task. In some cases, you get a reward at each step to find out that whether you are making any mistakes.
Imagine you want to teach a robot to walk without getting stuck by hitting a mountain, but you will not explicitly teach the robot not to go in the direction of the mountain:
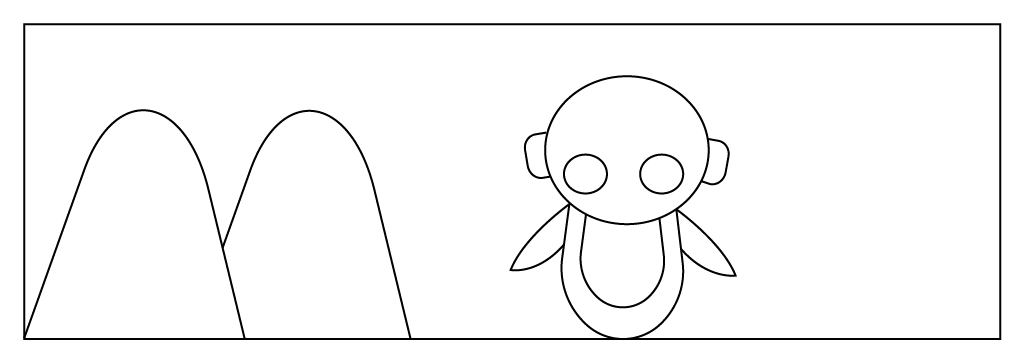
Instead, if the robot hits and get stuck on the mountain, you will take away ten points so that robot will understand that hitting the mountain will result in a negative reward and it will not go in that direction again:
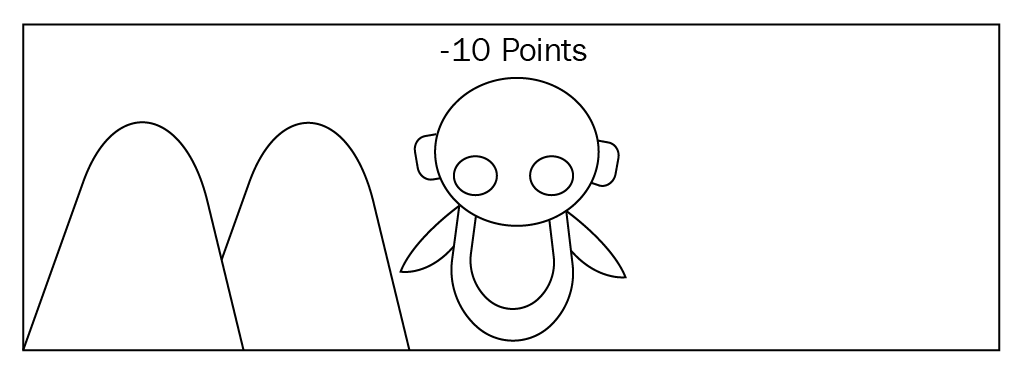
You will give 20 points to the robot when it walks in the right direction without getting stuck. So the robot will understand which is the right path and will try to maximize the rewards by going in the right direction:
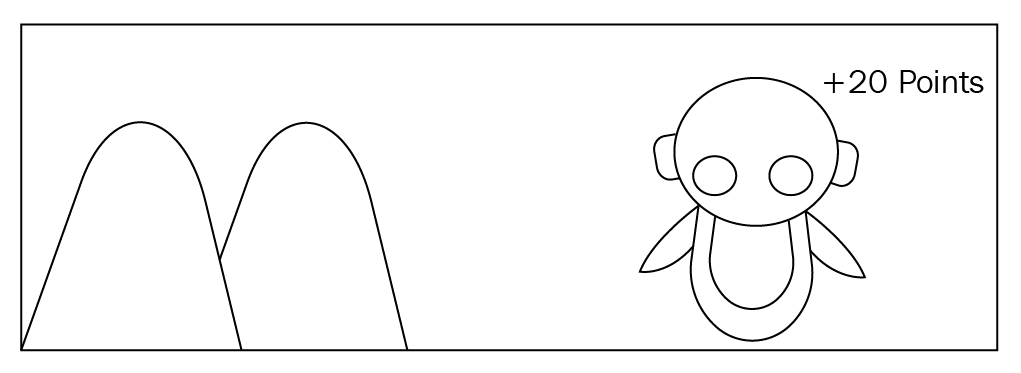
The RL agent can explore different actions which might provide a good reward or it can exploit (use) the previous action which resulted in a good reward. If the RL agent explores different actions, there is a great possibility that the agent will receive a poor reward as all actions are not going to be the best one. If the RL agent exploits only the known best action, there is also a great possibility of missing out on the best action, which might provide a better reward. There is always a trade-off between exploration and exploitation. We cannot perform both exploration and exploitation at the same time. We will discuss the exploration-exploitation dilemma in detail in the upcoming chapters.