




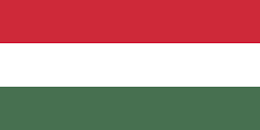

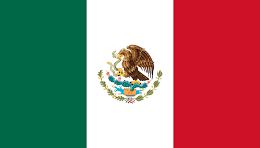


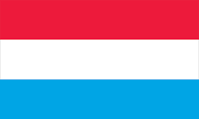



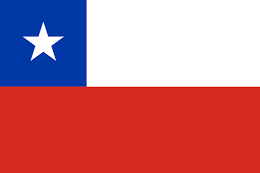



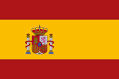


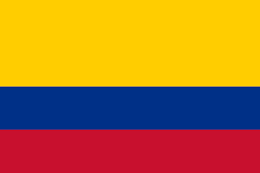
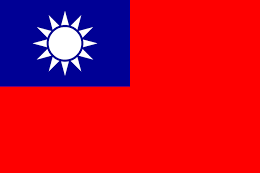


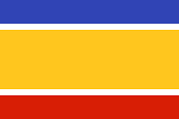
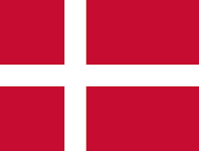
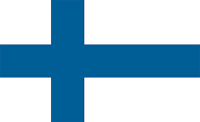



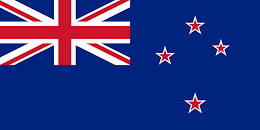


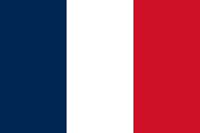



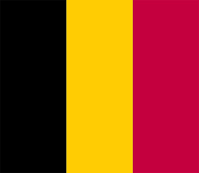

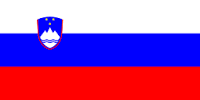




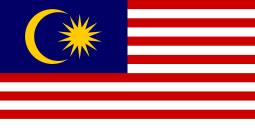


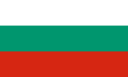




Researchers from Rochester Institute of Technology published a paper which describes a method to maintain speed and accuracy when dealing with high-dimensional data sets.
This paper titled Sparse Covariance Modeling in High Dimensions with Gaussian Processes studies the statistical relationships among components of high-dimensional observations. The researchers propose to model the changing covariances of observation elements as sparse multivariate stochastic processes.
Particularly their novel covariance modeling method used reduces dimensionality. It does so by relating the observation vectors to a subspace with lower dimensions. The changing correlations are characterized by jointly modeling the latent factors and factor loadings as collections of basis functions. They vary with the covariates as Gaussian processes.
The basis sparsity is encoded by automatic relevance determination (ARD) through the coefficients to account for inherent redundancy. The experiments conducted across various domains using this method show superior performances to the best current methods.
In many AI applications, there are complex relationships among different components of high-dimensional data sets. These relationships can change across non-random covariates, say, an experimental condition. Two examples listed in the paper which were also used in the experiments to test the method are as follows:
In such cases, the modeling methods used typically combine heterogeneous data taken from different experimental conditions or sometimes in a single data set. The researchers have proposed a novel covariance modeling method that allows cov(y|x) = Σ(x) to change flexibly with X.
One of the authors, Rui Li, stated: “This research is motivated by the increasing prevalence of high-dimensional data sets and the computational capacity to analyze and model their volatility and co-volatility varying over some covariates. The study proposed a methodology to scale to high dimensional observations by reducing the dimensions while preserving the latent information; it allows sharing information in the latent basis across covariates.”
The results were better as compared to other methods in different experiments. It is robust in the choice of hyperparameters and produces a lower root mean square error (RMSE).
This paper was presented at NeurIPS 2018, you can read it here.
How NeurIPS 2018 is taking on its diversity and inclusion challenges
Deepmind’s AlphaZero shows unprecedented growth in AI, masters 3 different games
Facebook’s artificial intelligence research team, FAIR, turns five. But what are its biggest accomplishments?