So, what did we do in RL? We tried to find the optimal policy given the reward function. Inverse reinforcement learning is just the inverse of reinforcement learning, that is, the optimal policy is given and we need to find the reward function. But why is inverse reinforcement learning helpful? Designing a reward function is not a simple task and a poor reward function will lead to the bad behavior of an agent. We do not always know the proper reward function but we know the right policy, that is, the right action in each state. So this optimal policy is fed to the agent by human experts and the agents try to learn the reward function. For example, consider an agent learning to walk in a real-world environment; it is difficult to design the reward function for all the actions it will do. Instead, we can feed in to the agents the demonstrations (optimal...





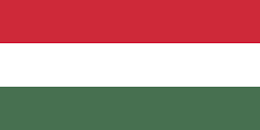

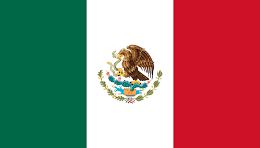


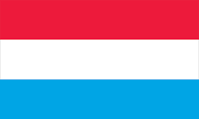



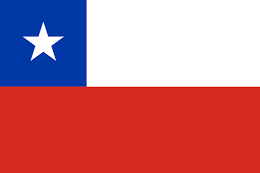



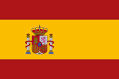


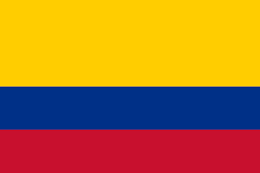
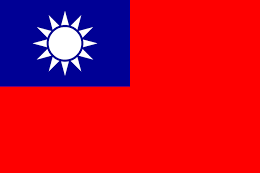


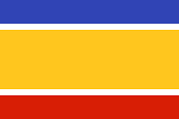
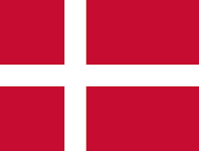
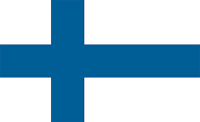



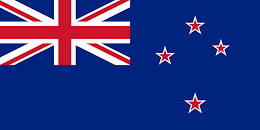


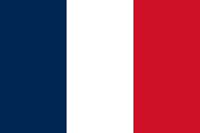



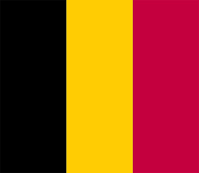

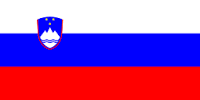




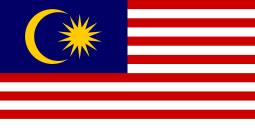


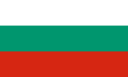



