A RAG-driven generative AI pipeline
Let’s dive into what a real-life RAG pipeline looks like. Imagine we’re a team that has to deliver a whole system in just a few weeks. Right off the bat, we’re bombarded with questions like:
- Who’s going to gather and clean up all the data?
- Who’s going to handle setting up OpenAI’s embedding model?
- Who’s writing the code to get those embeddings up and running and managing the vector store?
- Who’s going to take care of implementing GPT-4 and managing what it spits out?
Within a few minutes, everyone starts looking pretty worried. The whole thing feels overwhelming—like, seriously, who would even think about tackling all that alone?
So here’s what we do. We split into three groups, each of us taking on different parts of the pipeline, as shown in Figure 2.3:
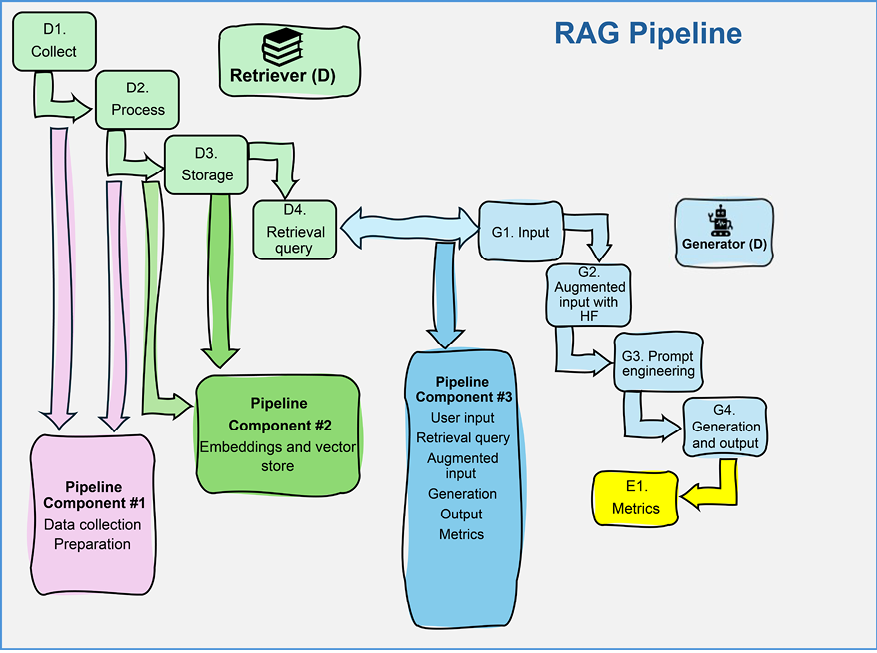
Figure 2.3: RAG pipeline components
Each of the three groups has one component to implement:
- Data Collection and Prep (D1 and D2): One team takes on collecting the data and cleaning it.
- Data Embedding and Storage (D2 and D3): Another team works on getting the data through OpenAI’s embedding model and stores these vectors in an Activeloop Deep Lake dataset.
- Augmented Generation (D4, G1-G4, and E1): The last team handles the big job of generating content based on user input and retrieval queries. They use GPT-4 for this, and even though it sounds like a lot, it’s actually a bit easier because they aren’t waiting on anyone else—they just need the computer to do its calculations and evaluate the output.
Suddenly, the project doesn’t seem so scary. Everyone has their part to focus on, and we can all work without being distracted by the other teams. This way, we can all move faster and get the job done without the hold-ups that usually slow things down.
The organization of the project, represented in Figure 2.3, is a variant of the RAG ecosystem’s framework represented in Figure 1.3 of Chapter 1, Why Retrieval Augmented Generation?
We can now begin building a RAG pipeline.