In this chapter, we were introduced to the TRPO and PPO RL algorithms. TRPO involves two equations that need to be solved, with the first equation being the policy objective and the second equation being a constraint on how much we can update. TRPO requires second-order optimization methods, such as conjugate gradient. To simplify this, the PPO algorithm was introduced, where the policy ratio is clipped within a certain user-specified range so as to keep the update gradual. In addition, we also saw the use of data samples collected from experience to update the actor and the critic for multiple iteration steps. We trained the PPO agent on the MountainCar problem, which is a challenging problem, as the actor must first drive the car backward up the left mountain, and then accelerate to gain sufficient momentum to overcome gravity and reach the flag point on the right mountain...




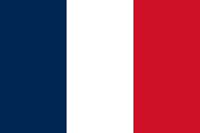


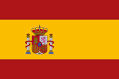



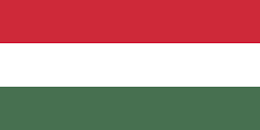

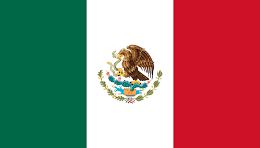


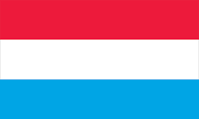



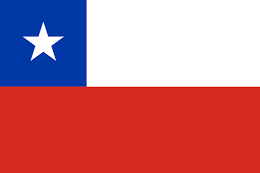


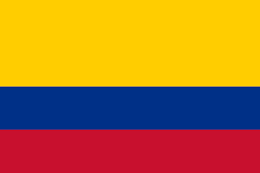
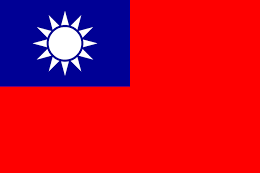


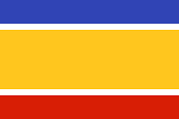
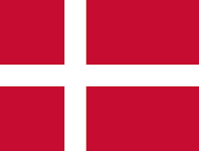
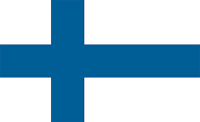



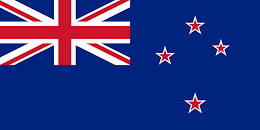





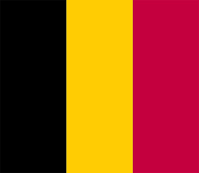

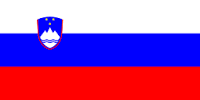




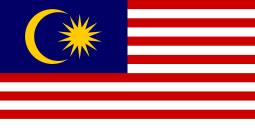


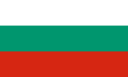


