We will first learn about TD learning. This is a very fundamental concept in RL. In TD learning, the learning of the agent is attained by experience. Several trial episodes are undertaken of the environment, and the rewards accrued are used to update the value functions. Specifically, the agent will keep an update of the state-action value functions as it experiences new states/actions. The Bellman equation is used to update this state-action value function, and the goal is to minimize the TD error. This essentially means the agent is reducing its uncertainty of which action is the optimal action in a given state; it gains confidence on the optimal action in a given state by lowering the TD error.




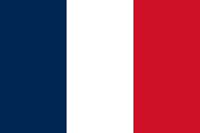


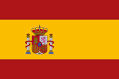



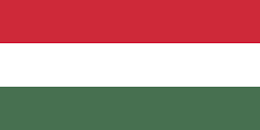

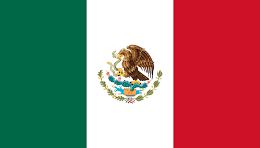


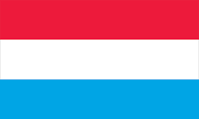



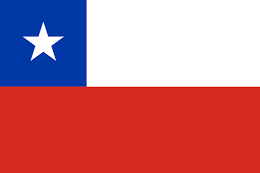


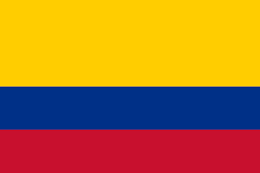
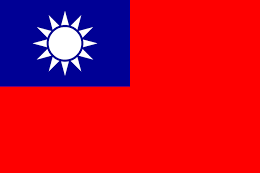


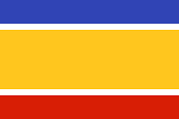
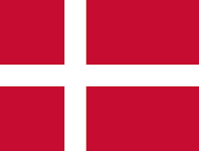
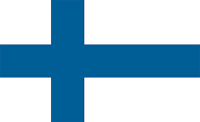



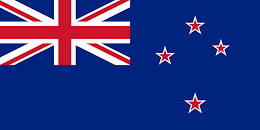





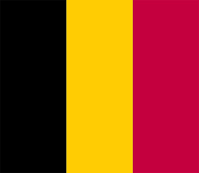

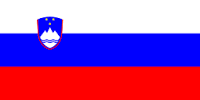




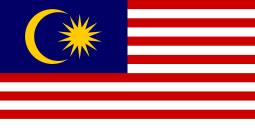


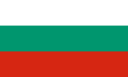


