In this project, we have explored what a reinforcement algorithm can manage to achieve in an OpenAI environment, and we have programmed a TensorFlow graph capable of learning how to estimate a final reward in an environment characterized by an agent, states, actions, and consequent rewards. This approach, called DQN, aims to approximate the result from a Bellman equation using a neural network approach. The result is a Lunar Lander game that the software can play successfully at the end of training by reading the game status and deciding on the right actions to be taken at any time.




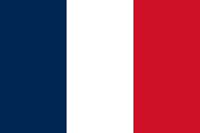


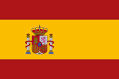



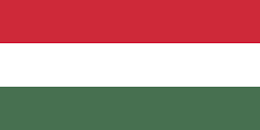

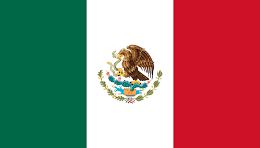


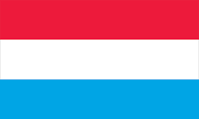



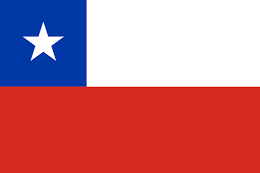


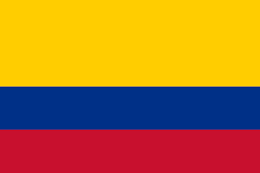
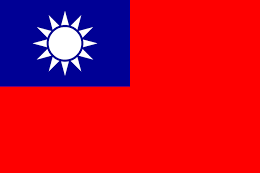


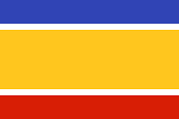
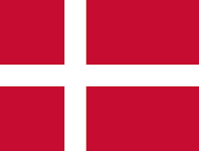
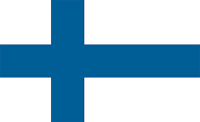



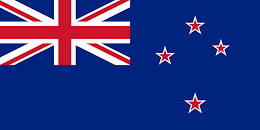





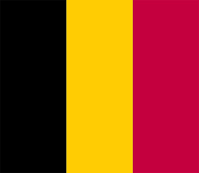

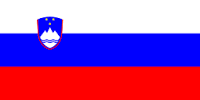




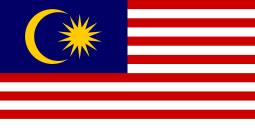


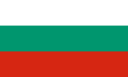


