Introduction to deep learning
Deep learning is a class of machine learning algorithms which utilizes neural networks for building models to solve both supervised and unsupervised problems on structured and unstructured datasets such as images, videos, NLP, voice processing, and so on:
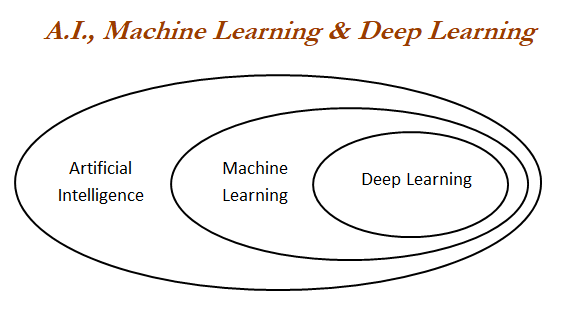
Deep neural network/deep architecture consists of multiple hidden layers of units between input and output layers. Each layer is fully connected with the subsequent layer. The output of each artificial neuron in a layer is an input to every artificial neuron in the next layer towards the output:
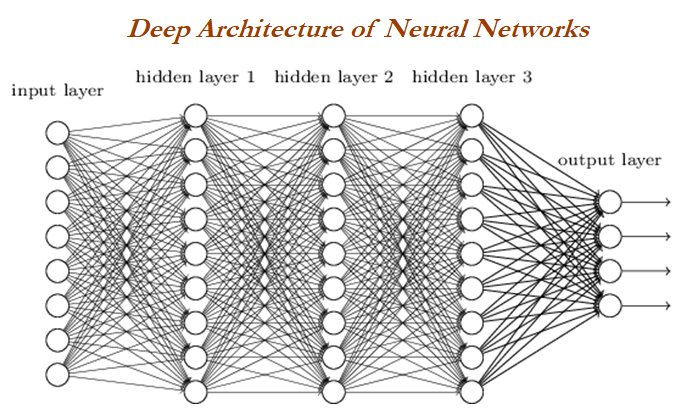
With the more number of hidden layers are being added to the neural network, more complex decision boundaries are being created to classify different categories. Example of complex decision boundary can be seen in the following graph:
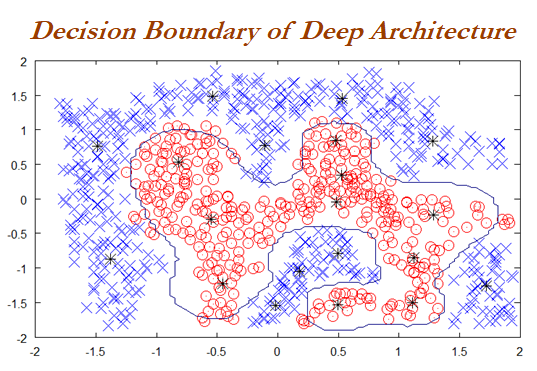
Solving methodology
Backpropagation is used to solve deep layers by calculating the error of the network at output units and propagate back through layers to update the weights to reduce error...