Data preparation
The trading experiment is tested in a cryptocurrency exchange called Poloniex. In order to test the current approach, m = 11 non-cash assets having the highest volume are pre-selected for the portfolio. Since the first base asset is cash, that is Bitcoin, the size of the portfolio is m+1 = 12. If we had tested in a market with larger volumes, such as foreign exchange market, there m would be as large as the total number of assets in the market.
Historical data of the assets is fed into a neural network, which outputs a portfolio weight vector. Input to a neural network at the end of period t is a tensor
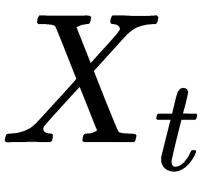
, of rank 3 with shape (f, n, m), where:
- m is the number of pre-selected non-cash assets
- n is the number of input periods before t (here n = 50)
- f=3 is the feature number
Since n = 50, that is, number of input periods is 50 and each period is of 30 minutes, the total time frame = 30*50 minutes = 1500 minutes = 25 hours. Features of the asset i on time period t are its closing...